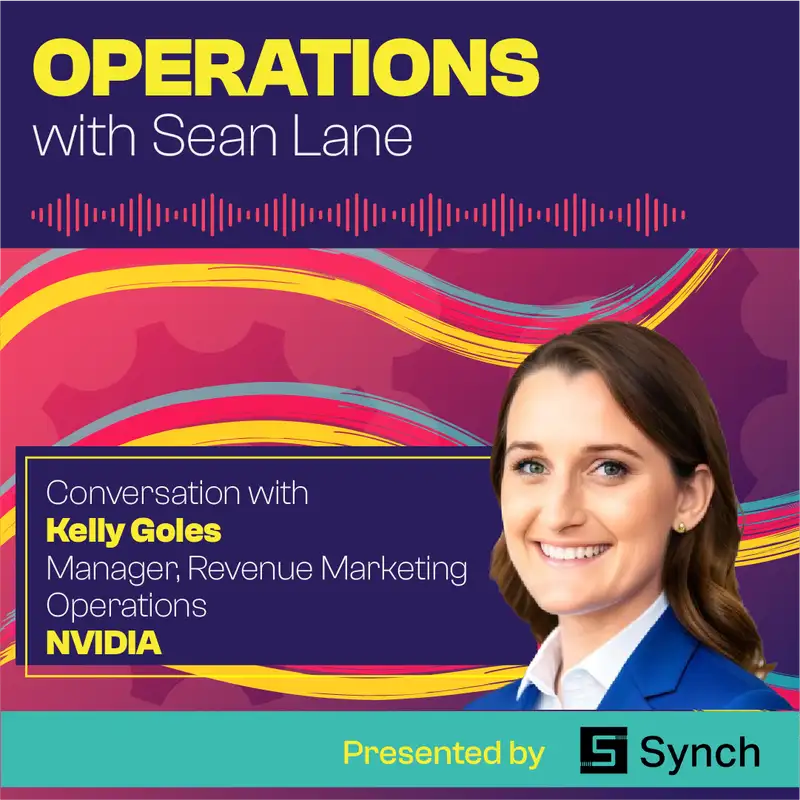
Accelerating Commercial Performance through AI with NVIDIA's Kelly Goles
Sean Lane 0:01
Yes, sales and rev ops leaders find themselves drowning in a sea of multi vendor tech stacks. The result less time selling more time managing tools. Synch is flipping that script. Cinch platform leverages AI to minimize the operational burdens of running your revenue organization, their AI admin completely changes the way that you build and maintain your Salesforce instance, so your team never has to build a flow, a dashboard or an APEX trigger ever again. No consultants or additional headcount required. And synch doesn't stop there. They've unified all your go to market reporting with a shared Analytics Suite, forecasting, deal review, pipeline management, alerts, lead assignment, all at your fingertips with zero implementation time. The best part, all of cinch is reports and insights. Talk to each other, sharing data to optimize your sales process automatically. Cinch isn't just a tool, it's your revenue. Co pilot, learn more and schedule a personalized demo today@withsinch.com that's W, i, t, h, with cinch, S, Y, n, C, h.com,
Hey everyone, welcome to operations, the show where we look under the hood of Companies and hyper growth. My name is Sean Lane. If you've had any conversations about AI or the big market players in the AI space over the course of the past year, chances are you've at least heard the name Nvidia. In simplest terms, Nvidia makes really powerful chips that help computers perform really complex tasks, especially those involving graphics and artificial intelligence. What's something like that worth? Well, their current market cap is $3.2 trillion and they just wrapped up fiscal year 25 with $130.5 billion in revenue, crazy. And look, I was a communications major, so I'm not going to sit here and try to break down the amazing technological advances behind Nvidia's chips, but I do appreciate a stellar rev ops team when I see one, and Nvidia has had a bona fide Reb op star on its team for the past six years in Kelly goals. Kelly is the manager of revenue marketing operations at Nvidia, and I'm not exaggerating when I say Star Kelly literally won lean data's 2024, ops Star of the Year Award at their annual conference. So she was someone that I really wanted to talk to. Kelly and her team have been tasked with figuring out how to use AI to accelerate commercial performance, and that's exactly what they've done through her team's work, Nvidia's go to market team has saved 1500 hours per week of sales users time, and they've 4x the amount of leads that reps can work. In our conversation, Kelly and I talk about how her team thinks about the reality of AI agents. We talk about how she focuses on scaling with operations versus scaling with head count, and where the responsibility of AI experimentation and enablement ultimately falls at Nvidia. To start though, with such a spotlight on the company, I wondered if Kelly felt pressure to be on the cutting edge of how they use AI internally. I
Kelly Goles 3:24
would say it's not a pressure. I would say we're all excited about it, and I would say we're all excited about it, and we're super lucky, because we have every resource in the world to kind of be able to execute and actually do what we're thinking about. So I would say, like we've, we've been looking and because we're an AI company, we talk about certain concepts. We talk about ways to use data. And since rev ops, the area that we're in, it's a data heavy workflows and teams and and we're oriented around that, we see the possibilities of it. So I wouldn't say it's as much as like a pressure, but at that I think, in terms of us being like a well oiled machine as we work towards some of these things, I think we have the same challenges, and we've gone through the same things that every company has probably that's trying to implement some form of AI, or work with other third party vendors on implementing what they've created for AI. So I think it's probably the same challenges. I think for us too, it's an interesting thing, because we've reinvented some of our go to markets, and we have different ways of selling so for example, like we've been primarily hardware company, now we're starting to sell more software. So as we transition with some of those things, we probably have similar growing pains to companies that are also maybe selling software. In the recent amount time we have been to
Sean Lane 4:35
I love that. I love the way you flip that. And it's the opportunity that's in front of you all, as opposed to and pose to the pressure. So let's talk about some of those opportunities. Right To your point, you and everyone else for the last year or so has been trying to figure out not only what's possible, but then kind of what's the right mix of what's possible for you and for your go to market. And so can you take me through kind of how you have been kind of exploring. And experimenting with some potential use cases that are actually going to be a good fit for your go to market at Nvidia,
Kelly Goles 5:08
yeah, I think that we approached it similarly to a lot of peers. And I think it's been happening. You know, within the last year or two, people have been talking about how rev Ops is kind of primed for, like, a AI transformation. I think it used to be called, like, digital transformation. That was, like the word, and now it's aI transformation. So like, every martech tool is now like a platform. They all have some sort of AI offering. So I think one of the ways that we approached it was, okay, we're already partnering with, like, a lot of these vendors who are coming out with some sort of AI feature. That's like, try to work with them and test it, or partner and see, like, what they're thinking, what it's capable of. I think the other thing that we did, we started looking for point solutions. So we started being, like, looking for ways that we could do smaller scope projects. So for example, one thing for us, we were like, Oh, well, we have, like, a lot of junk leads that come through from, like, our web forms or, you know, various things like that. Maybe like this would be a way that we could automate and test and run like a project on that which we did. So we started looking at like different point projects and solutions and almost forming like these, POCs for some of these, like smaller scale projects. And I've heard people do similar things too, like crawl, walk, run approach. And so I think we did the same thing, like, let's look at our existing vendors that were coming out with things, and then let's look for more like point solutions. It's kind
Sean Lane 6:28
of interesting that, like, if you look at the last few years, you know, we kind of went from this very point solution heavy world where all of a sudden people started to get, I think, a little bit more platform focused and like 2021, to 2023 and now, all of a sudden, to your point like, because there's so much now that's possible point solutions, it feels like might end up becoming something that people are paying more attention to, because you can go and attack this very specific problem with a new tool that wasn't possible or available before. And all of a sudden, like you have another opportunity. Is that the way you guys are thinking about it, because, like, the tech stack evaluation at that point must be pretty intense, yeah. I
Kelly Goles 7:07
mean, I think that's how we started with it. So I think most people, like, they're approaching it to your point, like, when we have the automation, digital transformation thing happening, people will start solving for point solutions. Now we have this new AI thing. So people it's either point solution or better way framing is probably smaller project in scope to try to, like, figure out, like, how can we start using it? Because if you start thinking about, well, how to re architect everything and automate with AI and plan, and that's a way bigger project than like, hey, let's start small with, like, a couple things and figure out, like, what's working for us, what's not. So we started down that same path, I would say, as everyone did, then we kind of moved into, like, the building phase, and it's like a more like, how do we group some of those smaller projects or point solutions, what they were offering, and group it into something that's more like, elevated to our platform that we were building out? So yeah, we've been consolidating in terms of traditional, like, martech stack too, right? Like, how do we move away from point and how do we like approach things with one platform? And I think the AI projects was the same. We started with these smaller ones, and then now it's like, well, how do we actually make change on the platform that we've been building out with our martech stack,
Sean Lane 8:12
regardless of whether you're a firm believer in platform first or you always want the best in breed point solution, Kelly's point is that she's focused on finding ways to solve specific problems or points of friction faced by her team at Nvidia. I asked her if she could take us inside the company and walk us through a specific example. Here's
Kelly Goles 8:33
Kelly. So I think, like, same thing with when we think about the crawl, walk, run. I think we all have the vision of the run part at the end, which is like, and I think a lot of people are talking about now, is this idea of like an AI agent. And Salesforce launched their version at Dreamforce in a big way. And that in video, we look at AI agents as something similar, too, like we have other products that are coming out around this AI agent that will help them be more like this assistant based thing. So we kind of work backwards from there, because I think one of the things with AI agent is they're, in a sense, automating the operating model that you've already built out, and they're working off of the data that you have. So we started looking at, okay, if we want something to automate, something to work on this front beyond do we have even, like, the right data for them? That was, like, one key thing that a lot of people, I think, are starting with too, which is, how do we have, like, the right data and any gaps around things that we would train on, or things that we would automate off of? So like, one big one for us, like this year as even an example, we brought on people.ai that was, like, a big shift for us and kind of a game changer. We realized that we have a lot of sellers that talk to a lot of different contacts at their accounts. They're engaging in a lot of multi threaded activities with meetings and emails and phone calls. I mean, all of that data wasn't necessarily getting written back into CRM. We're like, a usable data source. So like people that I've been a great partner for us and like trans. Forming and basically automating all of that data capture and putting it into a format for us at Salesforce, then we can actually use for a lot of like these Gen AI predictive projects then that we have going. So we started shoring up things like that and kind of working backwards from the end state. And then, like, how do we build up the operating model? How do we have, like, the right data to do all that? And I think even like as Al professional since we've been moving it over to your point, like a platform or automations over the last couple years, all of that is still going to be so key as people start to look at like an AI agent, or anything like that, because the agent is still executing under the operating model that you built, right? So if you don't have a good operating model, you don't know what you want the agent to actually do it's not going to be like, a successful outcome. Then for, I don't think for what you're looking for, and
Sean Lane 10:46
that's even more true about the data building blocks that you just described, right? If you've got all of these insights that you want to drive based off of, you know which contact roles are associated with opportunities, but no rep in the history of reps wants to associate those contact roles with their opportunities, then, like, good luck, right? But you've now found a gap in terms of your use of people AI to both identify who they're talking to, but then also, more importantly, to bring those back into the system to use for whatever future insight or workflow that you want to create. How do you kind of work cross functionally with your different partners internally to make sure that, like, if you are tackling a new problem, whether you're using AI or not, honestly, that you have all the right kind of data there to be that foundational layer for whatever it is you end up going to build later. Yeah,
Kelly Goles 11:34
I think it goes back to some of, like, the first principle stuff around, like, you know, at the end of the day, we're still servicing sales. We're still servicing like real people. We're still trying to solve and accelerate their workflows. I don't think AI is going to replace, for example, like our sales team in a large way, or anything like that. So I think when we cross functionally work with teams, we're more like around solving for something that they're already working on to try to help accelerate sales. So for example, like customer success, if they're already trying to look at like a churn model, for example, they have a good idea, like that customer success team, or that customer success ops team, of like, what would cause someone to turn for like, the product that they're selling, what wouldn't and so then we would partner with them and say, Okay, well then if you need to have this kind of outcome, so then our customer success team can go out and sell like, let's work backwards then and see like based on what you're seeing and those variables like, how can we build that data in? So I still think we keep it very people oriented and workflow oriented, and work with those people on that way, and then work backwards on like what we need.
Sean Lane 12:39
I appreciate that Kelly and her team are so laser focused on their internal customers, the end users for what they're building. They are building to solve specific problems for those users, regardless of whether AI is powering that new potential workflow or solution. And she stresses that we can't just jump ahead to the end run state, we have to crawl and walk first, which sometimes might mean some pretty painful foundational data work. And none of that is new. This has been the type of work that operators before AI were already doing. So what are Kelly and her team doing differently now that the tools available to them are changing.
Kelly Goles 13:20
So my whole rev ops team, we've basically been trying to help orchestrate and automate everything for these different sellers and these different workflows in our day jobs now. So for example, we have lean data, and they do the routing. We have a sales engagement tool like sales locked outreach sales, for sales for sales engagement, different things like that. We have lead scoring, where we're basically trying to say, like, Hey, these are the right people we're going to orchestrate and route to those customer success, yeah, people, or the account manager, or for us, there's like a team of four, so it's like XYZ number of people reaching out to an end customer. And we're planning out that logic and saying, like, you know me, Kelly, I'm going in building my routing rules and saying based on this industry and this attribute about this person, we're going to route it to this person, and then we're orchestrating the follow up and saying, and then they should trigger into this sales engagement sequence with this type of content and this type of follow up, and we're kind of manually doing all of that, but that's where I Think the AI agent can come in and actually say, learn and react and build out things better than we can, because we're basically manually doing it. I see the AI agent coming in and automating all that in a way, and that's where I'm big on saying that even all the work that ops teams are doing right now in terms of building out that operating system, that's so key, because that's what AI can come in. I think it helps accelerate it and work through. But if you don't have a good idea of, like, some of those manual things that you're putting in right now, what outcomes that you do want at the end, I don't think it's going to be a successful deployment then either.
Sean Lane 14:52
I mean, it sounds like just as much of this work, you know, while it's focused on kind of those end users, those. Customers that you have internally, right? The salespeople, it sounds like just as much of the benefit and potentially the change in the type of work is going to come to the people on your team, right, the people who will actually be the ones both kind of designing how these tools are implemented, but then hopefully there's less kind of like maintenance and tweaking, right? Because in the example you just described, one, like the person who's designing all of those routing and like automation decisions, needs to have a pretty in depth understanding of how the business works in order to do those things right. And then also, they need to be able to go back, you know, whatever, 3060, 90 days later, and then make all of the manual tweaks to the routing, to the automation, to the enrollment, whatever, based off of things you're learning within the business. But now I think based on the kind of newer world you're describing, some of that, like maintenance and later tweaking from ops, people might be removed. Am I thinking about that the right way? I think
Kelly Goles 15:52
so. And I also think it will be better at getting the right people to sellers with the right part. Sure, for us, it's like a lot of AB testing, and it's kind of like, send it out, and then we'll see after you do it, or we'll kind of do that. And we learn as rev, if you look at a company like Nvidia, for example, like we shelled every industry, we have 1000s of products to use that we sell, the messaging can be so different. So to have, like, even a team to go in and kind of manage and looking to do all the things we're trying to do, if we were to go down the manual route, not like that, AI agent route, it would be so many hours and a team of people were probably working on it. So it's a scaling way, I think, for us also,
Sean Lane 16:28
yeah, I mean, I know you and I have chatted about that a little bit, and just like, kind of your charter of how you can scale through some of these tools, as opposed to just by adding more people. Like, how do you think about that mindset internally? Right? Because, going from the version that you just described with the manual creation to the brand new version, that's like, kind of, like, this optimistic future, you know, that doesn't happen overnight. And everyone talks about, like, the really cool use cases that are possible, but then to actually pull it off across such a breadth of product lines and customers and industries like, that's really hard. So like, how are you going to do that? How are you doing it right now? Yeah,
Kelly Goles 17:06
I think it comes down to like, what we talked about, too, the platform versus like, point solution. I think we're in this point of consolidation because, like, let's just say you do want to have an AI agent that's orchestrating across everything, either a they're going to have to orchestrate across all of your existing martech stack. So they're gonna have to work with, like a sales lock and lean data and all these other ones, and kind of plug in, or you move towards, you have one sort of platform, which I think was just what Salesforce is selling to, right of like, you do everything in Salesforce, then the agent sits on top of it, and it's an execution platform, versus Salesforce just being like a data layer and everything else that's third parties that's on top of it. So I think for us right now, we're still making on that operating model. We're still trying to figure out, like, how's the best way to have like that AI, agent, piece and component come in and work with the sellers, or work with us as opt so I think we're still trying to figure that out too. But the best thing that we can do right now, and what I suggest people is figuring out what that actually looks like, and evaluating, like, what your go to market is, what your teams look like, what are they using? How are they interfacing? And then figuring out from there, like, what the best way to move forward is? Because, like, some tools I've seen with AI agent, it's like there are whole other platforms now you've incorporated, like, another tool and another interface. Or do you want to try to double down and use your existing interfaces like a Salesforce? Because that's best for you or not? So I think it's kind of like an exercise you have to evaluate for yourself.
Sean Lane 18:29
If you go back and listen to episode 134, of our show with Brett queener from bonfire ventures, you'll hear him echoing a lot of Kelly's same questions about where and how we will all inevitably interact with these different AI interfaces. Will it be one platform like Salesforce with agents plugging into an already existing data set, or will we have a number of different interfaces that are separate but pulling from all the different tools in our tech stack? I think that very much remains to be seen now, Kelly, because she works at Nvidia, and because she's put so much thought into this work, is someone that people turn to for advice on this topic, and through working through it herself, she's codified a three step process for how to transform your go to market strategy using AI. Her three buckets are one triggering so how prospects enter your funnel in the first place and demonstrate intent. Two, orchestration, routing the right prospects to the right people. And three, engagement, how your team will ultimately try to contact those people. I asked Kelly to take us through those steps and how she makes them come to life at Nvidia.
Kelly Goles 19:39
I'm big on those three buckets, because I think it's like, how we've set up even automations in general. And I think, like, that's also, if you had, like, an AI agent come in, they'd be operating in those three buckets, and the triggering. What I mean by that is like finding the right accounts and the right people at the right point in time. So I know we're super lucky, like we have a in house data science team. Um, thank you, Santa Paul and everyone, if they're listening, they've actually gone in and done some things too cool around, like building out like a custom intent model. So they actually look for things of our own internal first party triggers, so like engagement, like on our website, or engagement with our activities that we can track internally to Nvidia, like developer downloads of things or different triggers and touch points like that. They also leverage third party data, so things like six cents, or things like intensify or other vendors, and they aggregate it, and they basically have been helping us say, like, Okay, this is the right people that look like they're in market to reach out to. And so once we have like, those people identified, then we can actually queue off different engagements and interactions that way. So the orchestration component, that's where it's like now we've identified this person's ready for something. We kind of know what they might be interested in. We know what they could want to talk about next, and now it's actually write them to the right team member. Maybe it's customer success, maybe it's an account manager. Maybe it's someone who's on our, like, Developer Relations team. So that's like the orchestration piece of like, now, who do we route it to? And then the final piece is the engagement component, which is like, well, what are they actually supposed to talk to them about? What does that talk track look like? And what's like the right assets and collateral that they can use to help what that person is interested in? So I think, like, the triggering the right people at the right time, orchestration, routing it to your right person at Nvidia for us that should be following up, and what should they actually be following up with? That's kind of like the loop that we try to do. I think it's hard, because I think people get really into dashboarding too. And like looking at reports, and it's like, you give a seller like, Hey, check this report daily and see, like, what, which of your accounts are surging or, like, you know, these are the ones that are showing really high intent, and then try to go do something with it. So I think, like with this operating model, we're trying to do is, like, take out any of like, the manual guesswork and sort of queue up actual execution with that model versus just insights.
Sean Lane 21:59
And when you say, queue up actual execution. What do you mean by that? I
Kelly Goles 22:02
think I mean making it easier for sellers, not just to say, like, look at a dashboard and say, Hey, this is super nice. These are all the accounts that are surging. Let me think about that. As I continue to do what I was planning to do for the day, maybe I'll like do something with it. What I mean by queuing up actual execution? It's kind of going back to, like, that sales engagement component too, which is, like, here's the insight we built it into the process that we already have. And for most people will probably be like, lead scoring or whatever routing they're doing, and then whatever sales engagement tool we're actually building it in to that. So then, if I'm a seller, I'm not looking at a dashboard, I'm looking at my task list of things I have to do when I log in, and it's kind of already queued up for me. Oh, I need to send this email to this person, because they're showing intent, and this is the talk track, so it's kind of queuing things up for them to take action on, or even in some cases, doing it for them, versus sort of this insights, and then forcing them to kind of think about it and go find the assets, go find the talk tracks, and then take action off of it.
Sean Lane 22:56
Yeah, it's so interesting, like choosing that moment. What is the right time to kind of bring that rep into the fold, both to either approve, you know, something that's already kind of prepped and ready to go, for them to go out the door, or for them to kind of craft on their own, like that. Question is tough, right? Because I would think about it like, up until pretty recently, I would say, if you had some sort of like Target account type strategy, you could say to your team, look like, this is the threshold from a fit perspective, and then this is the threshold from a behavior or intent perspective. And when it clears both of those thresholds, I'm going to give it to you to kind of take action, right? That's the best use of your time, knowing that this is a company that's a good fit, and they've shown some level of intent, right? And so your job, you know, Mr. Or Mrs. Rep, is to start from a point of personalization to the people that I'm telling you, clear these thresholds. And so now, like that line, I feel like is just going to keep getting pushed further and further down the funnel where you can then say, Okay, we've cleared behavior. We've cleared fit, and I've already personalized it based on everything we know about this person. And like, you could either just ship that out the door to the person, or you could give it to the rep for them to approve. But like, these are the types of kind of I think, like, design questions that ops people are going to need to be making, leveraging some of the new things that are available. Yeah.
Kelly Goles 24:20
And it's like, it goes back to the thing that we talked about too, like scaling with head count, or scaling with operations, even, like an engagement tool, or like, we're queuing up like that. If you had one person and they you took out like half of their prep work to reach out to someone, you know, you could double their efficiency, or do something like that. But it is a question, I think also depends on, like, your model, if you're a smaller startup, and you're trying to, like, build out some of this and maybe your kind of customer base, your leads coming in, the volume isn't too high, but when you look at something like for us, our thing is about scale. Because we have so many leads, we have a lot of different accounts that we're targeting, it's pretty large scale of what we're doing. So this, for us, is almost. In a coverage model too, because otherwise we won't be able to spend maybe as much, like personal one on one, maybe a lot of people are showing high intent, but we can't, kind of reach out to everyone. So this is like a way to automate
Sean Lane 25:10
that too, and because I know your focus is very much kind of top of funnel lead management type work like, what is the measurement for you that tells you whether or not some of these experiments are working. What are you looking at to see whether or not you're creating the leverage or productivity or conversion rate change that you think you're making? Yeah,
Kelly Goles 25:29
I would also say that it's top of funnel. But it's also the big shift for my team too right now is we're thinking of leads as also our existing contact and customer base. So it's the upsell, it's the cross sell. Everything that we're building out right now, it's more of like a sales outbound motion. And that's not limited to just inbound, like net new names, like coming through the lead nurture, especially if you think about for Nvidia, for example, like you bought the hardware, and then now we have software products coming up. That's like a big opportunity, I think, at least for our team to help accelerate, like some of those newer types of outbound motions that we have. So I would look at it just as the inbound like lead motion. I think it's how do we nurture the entire contact base of existing people, even though we already have and then you start layering into because it's not just sales too, like again, it's customer success, it's developer it's like all these other people, and for us also it's channel partners. So it's co marketing and CO selling, because that's like how we go to market. So it's like layering and all those people too. So sometimes it's not just like a direct sales motion, it's like some other sort of like nurture happening also,
Sean Lane 26:35
did you catch that? Did you write that down? Kelly's title is revenue marketing operations. And when I implied that that meant mostly top of funnel work, she jumped right in and expanded the definition of her team. Sure they're helping SDRs prospect better, but she realizes that everything her team is building is also perfectly applicable to post sale, cross sell and expansion motions so many marketing ops teams or marketing teams, for that matter, don't do this. This org design pairs really well with a company like Nvidia, because they have so many different product offerings. But executing work like this across pre sale post sale isn't easy. There are a lot of cross functional stakeholders that you need to bring along. So I wanted to know how Kelly and her team pulled that off. Yeah,
Kelly Goles 27:25
I think that's where the operating model that we built out is, like, shining is, for example, like, if everyone's working out of the same sales engagement tool, and you're triggering where most of those people like, what path they're going into in terms of engagement, you're kind of pre planning that all out together. So then we can partner, like, when we'll talk to Customer Success ops, like, when would we send someone to your team for this follow up, versus sending them to an account manager? And so when you build people into the tools, you have like, complete CRM base to like, with people.ai. Now for us, like mapping in when they're talking to customers, what they're talking to them about. It makes it pretty easy for us to trigger then off of all those interactions and, like, plan out where they should go. So that's kind of the benefit of us all being and working out of this third party tech stack too, and everything writing back to CRM, which I'm going to keep harping on, like, the data, but happily complete CRM. It's really kind of the only way to execute what we're doing, too. Yeah, I think
Sean Lane 28:22
that's so important, because it's so often the case that some of those engagement decisions are made by the individual teams, right? And so you end up with one engagement tool for kind of leads and new biz acquisition, another one that is run by kind of product for product communications, another that is run by the customer team, because they've got a different platform where they're measuring things like health, and so therefore they want to send their email messages from that, right? And then you've got a marketing automation tool kind of floating around on top of all of that, right? And so what you're saying is you've kind of managed to consolidate everybody to say this is the place from which engagement is sent out to any in any part of the customer journey.
Kelly Goles 29:05
Yeah, we're working on it, and at the very least you can see what everyone else is sending. That's been the key part for us, too. Like with the people.ai integration, it's like, you can go in CRM and see like, Kelly and Sean emailed today, and if I'm the the account manager, can see that and be like, hey, Kelly, since you're the internal Nvidia and on my team, can we talk about what you talked about, and then we'll kind of coordinate before we talk to Sean again. So the other thing too, it's all about the customer experience. It's pretty annoying too. Like, it's actually what started us down with people.ai path in the first place, is like our SDR and BDR team were reaching out to leads, new leads that came through. And they would reach out and they talk to them, and they'd be like, actually, we talked to someone on video for like, two years, and it was just that that account manager didn't put their contacts in. They were talking to them that kind of thing, because we didn't have that incentivized in our system that way. So it becomes like a better customer experience too, and we can pre plan everything out, versus kind of free for all.
Sean Lane 29:57
So what's up next for you guys? Right? You talked about all these different use cases you've explored. You're clearly been building this operating system internally. You know, where are you hoping to kind of go from here in the next 612, months, and what are the things you're going to continue to experiment with, with the team? Yeah,
Kelly Goles 30:16
I think we have this operating model that we've been building out. We're working on, we're AB testing, I think as we partner with, again, very lucky in house data scientists team, some of the things like, we start to look at the kind of bucketing project into two categories. I would say it's around, like, predictive and then the other buckets around, like Gen AI. So it's like, how do we do a lot of the forecasting, or, how do we do a lot of the predictions? Like we talked about of like routing the right people to the route at the right time, into the right cadences. Like, that's the kind of thing that we're going to be building out, almost somewhat like a AI agent, but basically our own prediction models mapping and building out that logic for us. So that's kind of one of the bigger things, I think. And then on the Gen AI component, it's also around things like, how do we write some of the sales emails? How do we maybe, do incorporate a version of, like a AI, SDR, and like an assistant, kind of where it's like writing the emails for them and then sending them their behalf, or reaching out to someone, maybe, like our leads that are maybe showing lower intent, some of our leads that are showing higher intent, just to get a touch point on them. So I think, like, those are, like the two buckets I think about as we go forward and
Sean Lane 31:26
on the Gen AI piece in particular, right? I feel like there's kind of two ends of the spectrum there, right? There's like a super controlled version where you have like, this centralized group of people within the organization whose job it is to figure out how the whole company should use Gen AI. And then the other end of the spectrum, it's like, everyone's kind of testing their own thing and figuring out Gen AI use cases that might be relevant for them and their role or their team. Like, how are you finding the right balance between that to both kind of cultivate the sense of like experimentation within the company, without having it end up in a world where, to your point, like, everyone's versions are siloed, or interactions are different, and you can't really create that cohesive customer experience.
Kelly Goles 32:09
Yeah, it's hard to do, right? Like, I think probably, like, our VDR team is probably using chat GPT since it came out, to write all their emails for the most part, like rewrites or things like that. But there are certain things in, like, the ways that we approach projects and worked with teams like at Nvidia, you can imagine, there's actually so many data science teams, so we have kind of, like a governing board of almost like projects, or different like ethics that we screen through, we mark, like, a lot of different projects. That's been helpful to, like, look through that, and then when it comes to Gen AI, like piece and like, trying to incorporate it. I think for us, the winner is going to be, how can we incorporate it into the workflow where, like, reps actually can write emails in a meaningful way? So I think it's going to be like looking at like that sales engagement component piece. For example, the two tools that we use right now are sales locked and Salesforce. Sales engagement. We're using running both in parallel for different reasons, but whichever one that we can plug into where reps are actually writing, so they don't have to go to an additional screen or additional things, and it can kind of be built in that way, and it actually can pull in data sources that are relevant. I think that's going to be the key, and incorporating enough data to aggregate to actually build it out too. So for example, I've seen different companies that pitch to us and they will write emails, maybe just based off of like one data source versus like. What you probably want is, you probably want to be super multi threaded. You probably wanted to incorporate things, for example, like our entire sales email base, of like, what emails are getting sent by your whole sales team, but you also probably want it to incorporate things like our nvidia.com, web pages, or for us, like high spot where you're keeping the sales engagement or enablement content, so different things like that that incorporate it. Because if you base it off of just one, it's probably not going to be as accurate, like if I base it off of what every sales rep is writing, it's probably good for the conversational tone, but they might be saying, you know, wrong things about something, or misquoting something, and so it can be referenced in a different way too. And
Sean Lane 34:06
are you, you know, this is a tough burden, I guess. But like, Are you sitting in rev ops, like the arbiter of all of that? That's a lot. Everything you just described is a lot right to figure out how it's working. You know, what's the best version of it for the team, like, does that fall on your plate? Is that a shared responsibility? How does that work? It's definitely
Kelly Goles 34:25
shared because there's so many teams trying to work on the same project. But well, we're responsible at the end of the day, it's for that sales enablement piece, and because we own all the tools that people actually execute out of it needs to integrate with what they're doing there. So I think that's the piece that we look at, like, at the end of the day, we're still looking at like, how do still looking at like, how do we enable sales reps to sell better? So we are definitely a part of the evaluation process and care about it being successful, because if it's not good, they won't use it, or worse, they use it and it's not good for our end customers. So I think like the burden of that kind of falls on us, especially if we're. Doing, like email plays, for example, we're coordinating all those, and we're sending out bad emails. We're definitely then like a key stakeholder. And we try to help where we can too. So even, like bringing on something like people.ai where it's an additional data source. Now, of like, all of the sales emails, we need to have that component built in. And then we work with the data science team like, Well, where else is the gaps? And what else do we want to bring in and what makes sense? And we bring sellers in too. We work with a lot of, like, sales leaders, and they help us give their input to since they have that knowledge of like, what's actually helping them. So we incorporate a lot of different opinions on it too. You
Sean Lane 35:40
room before we go. At the end of each show, we're gonna ask each guest the same lightning round of questions. Ready? Here we go. Best book you've read in the last six months?
Kelly Goles 35:55
I really like Shoe Dog, about the Nike co founder. That was a pretty good one
Sean Lane 36:00
for me. That's, like, one of my favorite business books of all time. Yeah, the recommendation for my brother. So that was pretty good one. That's awesome. Favorite part about working in ops. I
Kelly Goles 36:10
think it's like a big puzzle, and when you finally figure it out, and things kind of work, and it flows all together, that end satisfaction and knowing that you actually help people too at the end, it's pretty good feeling the
Sean Lane 36:24
best flip side, least favorite part about working in OPS, I
Kelly Goles 36:28
think sometimes it can be hard to do transformative work and ask people to go outside their standard workflows or operating processes. So trying to sell that vision and get people on board, taking all those opinions, it can be a labor of love sometimes. So I think that's probably the hardest part. That's
Sean Lane 36:48
a great answer. Someone who impacted you getting to the job you have today. Yeah,
Kelly Goles 36:53
I want to say actually, my dad on this one, he It's funny, when I started my internships too, like, he taught me this too, of like, like, when you're working on a project, he's like, try to think through. He's an engineer too, so it's helpful to think about, but he's like, try to think through, like, what the problem is through the whole solution, before you come up with an answer at the end. Try not to just kind of ask questions or start from the beginning, like, try to think through it in the end. And I feel like I still have that in the back of my head, and I still operate that way with a lot of projects that we work on, too. It's kind of almost like an engineer like engineering mindset to
Sean Lane 37:24
it. That's awesome. That's a great answer. Shout out to your dad, I'm sure, very helpful still to this day. All right, last one. Now your turn to give some advice. One piece of advice for people who want to have your job someday,
Kelly Goles 37:33
I think it's good to be data literate. There's two parts I think, to like this job. There's like the data and then the analytics component that you have to understand and enjoy, but then at the end of the day, you're kind of an internal salesperson too, so you need to be able to communicate clearly your ideas, the outcomes that you're trying to do, and be able to kind of persuade people to go on that path, kind of lead without authority. Might be a better way of saying it, or working cross functionally, I think, having that technical component, but then also that good interpersonal skills and communication is key to the success that I've seen here for our team too.
Sean Lane 38:15
Thanks so much to Kelly for joining us on this week's episode of operations, and congrats again on being the 2024 op Star of the Year. If you liked what you heard from Kelly and learned something from our show, make sure you're subscribed so you get a new episode in your feed every other Friday. Also, if you'd like to show leave us a review. Leave us a review on Apple podcasts or wherever you get your podcasts, six star reviews only. All right, that's gonna do it for me. Thanks so much for listening. We'll see you guys. You.