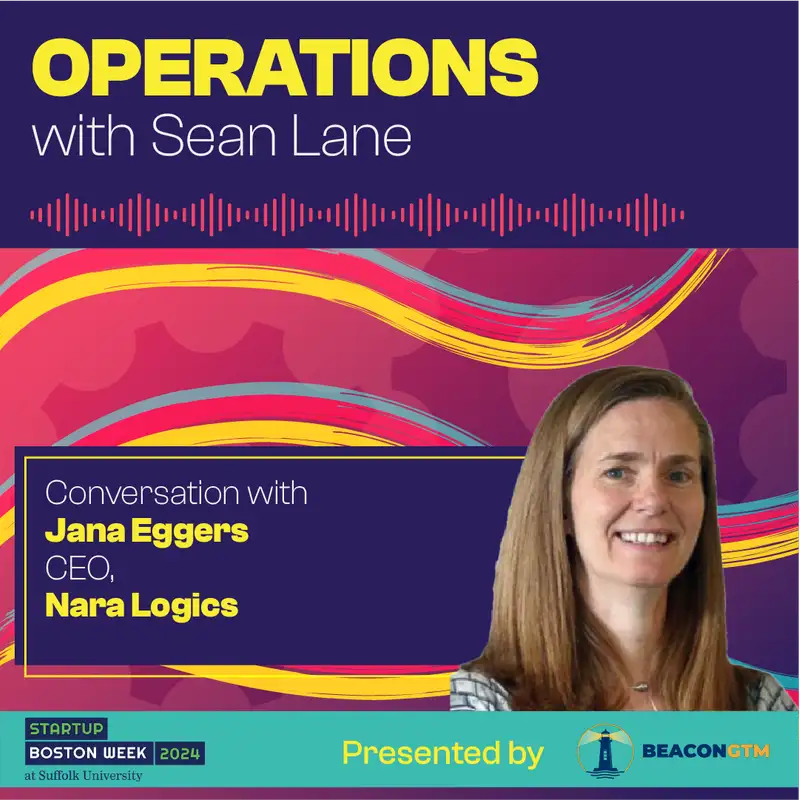
AI Is the Tool, Not the Answer with Nara Logics CEO Jana Eggers (Live from Startup Week Boston)
Sean Lane 0:06
Sean, Hey everyone, welcome to operations, the show where we look under the hood of companies in hyper growth. My name is Sean Lane. As we all know, AI is all the rage right now. It's easy to get caught up in the hype and just assume that AI is the answer to all of your problems. But in the midst of all this hype, it's important to remember two things. One, AI is not new, and two, AI is a tool to answer problems, not the solution itself. Now, per usual, I can't take credit for these insights on this show, those lessons were delivered via our guest today, Jana Eggers. Janna is the CEO of narrow logics, an AI advisor software company. And the reason I was so excited to talk to Janna is that she's an AI practitioner, but she's not new to the AI world. Like so many of us, her career has taken her from three person businesses to 50,000 person enterprises. She's done everything from opening the European logistics optimization software offices as part of American Airlines to natural language processing at Lycos back in 1996 to founding into its corporate innovation lab to get this researching conducting polymers at Los Alamos National Laboratory. I had the chance to catch up with Jana in person as part of our series of episodes from Startup Week Boston at Suffolk University, and in our conversation, Janna brings a healthy skepticism to help us separate the AI hype from the utility. We dig into what she sees companies getting wrong about AI and why she believes that AI augments human intelligence but doesn't mimic it. Also be sure to stick around until the end of the episode for one of my favorite Lightning Rounds we've ever done. To start, though, when I was prepping for this interview, something that Jana had written intrigued me. She said, quote, I support, subscribe and contribute to ethical, explainable and deployable. Ai, so my first question, naturally was, what does that mean? Well, I
Jana Eggers 2:06
think most people get the ethics. Unfortunately, as I was just having a conversation after the session with someone who said, how do we get more people to worry about the ethics? And I said, well, people are worried about the ethics at the high level. It's that bridge there. And so those three aren't separate. You know, the deployable ethics are really important. Like, how do we actually make them work in real life situation? And they're not just something posted on our website. What does it mean to them? And so the ethics part, I think we think we understand more than we do, probably a lot, like, revenue operations, right? We think we understand it a lot more than we do, which is why we need someone like you appreciate that. The second part of explainability is just wicked fun for me. So explainability can be as little as kind of saying, Okay, what are the major factors to this? Right? Which is a pretty easy analysis that's been around for a while. That's what I was using, you know, 30 years ago, and it's still valid these days. What we do is a bit more special than that. We're really tracing information all the way through to how it impacts the model, as well as how it impacts the answer. And so we call it explainability 360 which is, what if my signal didn't activate anything in the network, what activated something and then what on the answer side was unactivated? And it gets really nerdy as to why you need all of that. But if you have problems where the answer and understanding what drove that answer is really important, that's where we are. So we work in defense and intelligence, and you can imagine how important that is in there. We work in healthcare, and you can imagine why that's important. And then on the financial services side, it's all about regulatory so it's for them to be able to say, No, I know why this model is working this way, and what makes a difference. And then deployable. There's a lot of AI that's just in POC stage. And I'm not saying that's bad, that's awesome. People are learning about AI, but most AI projects fail like most startups fail. It's actually pretty close to the same number in terms they're both in the 90s, of projects that fail, and so we work with our customers, and I've worked throughout my career, is really focused on, how do I get this into production? How do I get people to use it? Even back at Los Alamos, where I was doing conducting polymer work, I was theoretician on the team. I was a mathematician, computer scientist, but I worked with chemical engineers, and they're really material scientists, and so they would ask me, I would come up with this beautiful model and say, Ah, this is the best conductivity you're going to get out of this. And they would go in and say, but why? And so that's when I got passionate about explainability. Is, how do I explain to someone why they should actually use the answer that I'm giving? And that's the only way you're going to get deployable.
Sean Lane 4:58
I would imagine, too. So the thing I had appreciated the most about our last conversation in preparation for today, and some of the things I've read that you've written or given interviews about, is that AI is not the solution. It is a mechanism to get to the solution, and I would imagine that being able to explain and deploy that mechanism then makes it easier once you do actually arrive at the answer to the problem, but that's just a means to get there. Am I thinking about that? Right?
Jana Eggers 5:25
Absolutely. I, you know, getting nerdy again, please. AI is really a system of systems thing. So it's one system that always fits within another, lots of systems. And you can have simple systems, like, when you look at a chat GPT or something, that's still a system. It's still taking some input from you, giving you some output. But that input part and that output part are not the AI. The AI is this one. So even in a basic situation like that, but normally you're looking to do something else with that. You're not just looking for that as a point solution on its own. So now you're seeing, how do we integrate chat GPT into dev environments, right for coding? And people focus on, oh, the chat GPT is doing the coding. It's giving some suggestions there, but there's a lot of other things around that. In terms of, how do I make sure it knows the right environment that it's writing code for? And my engineers regularly use it because they're looking for idea. It's kind of like their idea sparring partner, if you will, but for the code that they actually use to deploy, it's not usually that close to what chatgpt First did. It was more someone that's like, oh, did you think about this, or did you think about that? But the environment that they're going into, how it fits within the code that we have already like, you know what? Random next word generation? It's not random, sorry, but statistically next coding word generation does is different from what how we have coding standards within our organization. So that's what I mean by it's not gonna stand alone there. It's actually fitting in within a whole system. And our engineers have had to learn more rather than writing code, they've had to learn more how to read code. Is just one quick example, right? That's a change in the system that we're experiencing as a whole.
Sean Lane 7:19
And I think, you know everyone, and today, in this event, this week, is a great example of this. Everyone wants to participate in conversations like this, but I think so many folks have this chatgpt example as kind of a jumping off point. How are you teaching people to broaden themselves beyond that specific example that a lot of people have become very comfortable with very quickly, and be able to be comfortable in using the type of language you might use with a client or in a project, so that they can participate in these types of conversations. If chatgpt is 101, get us up to the 201, level. What
Jana Eggers 7:53
I love, and I was worried when chatgpt first got all of its big hype, and I'm like, Oh, this is gonna be a headache. And it's actually been wonderful. I actually even told my board this, this this morning, we had a board meeting, and I said it's actually been less worse than I expected, which says a lot. And the reason for that is, I think it's given us a common lane, a common experience. I shouldn't say language, it's because it's not language, but it's given us a common experience where we all like, had those answers that were like, Oh, how did it come up with this? It's amazing. And we've all had those like, and we've all had the, you know, middle of the road, so we've had this kind of common experience, and we can laugh about some of the things, and we can complain about some of the things. And we can be excited about some of the things, but it's given people more of an idea. Oh, AI in general might be like that too. It's not just chatgpt, it's all types of AI may have that. Wow, very bad, right? And so I think that experience so now I can more say, because most people before I could say things like, Do you ever use waves? You know how sometimes it's like, right on, but then other times it's kind of like, well, wait, they just shuttled me and everybody else off on the same street. And now this tiny little street is, you know, and so you can talk about that kind of thing, about the real timeness of AI and stuff like that, but they don't really connect with it because they didn't see the other options. But with chatgpt, they're often like, oh, it said this, what if I modify my prompt this way? Right? And so they're starting to learn more and be more interactive, rather than having a pretty hard shell on top of it that they can't quite penetrate into and really understand what's happening with the AI. It's
Sean Lane 9:46
pretty interesting that after decades spent as a practitioner in the AI world, even Jana found that chatgpt offered this new common experience through which we can all similarly understand the. Utility of AI, but remember, AI is the tool, not the solution, and Jan's mission is to contribute to ethical, explainable and deployable AI. So I was really curious, what did she mean when she said that chatgpt wasn't as bad as she thought it was going to
Jana Eggers 10:16
be. People have been less wowed by it than I expected. So I really did expect that people would give it more the benefit of the doubt than they did. So I'll give you an example. Kind of early on, I had a friend who said, Oh my gosh, I asked chatgpt to give me a day in I can't remember more in Italy it was, but she was going to Italy, and it just gave me this incredible day in Italy. And I was like, Well, did you do it? She was oh, well, I haven't gone yet. And then I said, Oh. I was like, Well, what made you think it was incredible? Well, it sounded good, right? And so, like, kind of as I peeled the onion, I understood more that she hadn't really questioned it. She thought it was magic. And what I've seen more is that people are questioning it, or they're asking things that matter to them, or they're asking it things that they're specialized in, rather than plan me a vacation in Italy where I didn't really know what I wanted, and I questioned it. And so I did ask her, I said, Hey, what about if you ask it to do the same thing for your hometown, and then see how you feel about it? And I was just curious. And she came back to me. She was like, You know what? She lives in New York. She was like, it gave me things that I could never do in one day. And she was like, Do you think I'm gonna experience that when I go and I was like, Well, you
Sean Lane 11:38
may. And this is something you've talked about a lot more broadly, which is just the idea that humans should be skeptical about the different use cases that they are experiencing with. Ai, can you talk a little bit more about
Jana Eggers 11:50
that? One of the pictures I showed was something that went viral six months or so ago, and it was a guy smoking in a McDonald's or something. And people were like, Oh, my God, can you believe this? And, you know, there was political, as you can imagine, overtones there of the type of person that would do something horrendous, like that. And if you looked at it, I mean even more than cursory, before you forwarded it, you could see the guy had six fingers, you know. I mean, anything you kind of look at for AI. Like, if you know AI, it's like, look at the ears, look at the fingers, you know, it's like, his shirt was, like, really kind of weird, like, it was half one kind of shirt and half another and, and it was really, really clearly AI generated, in my opinion, oh, like, it was a Coca Cola cup that looked like Coca Cola, but the lettering was totally off. It was scribbled, so there were things, but it was forwarded. I mean, the amount of forwarding that happened like, and that's what somebody was tracking, is look how forwarded and riled up people got about this, and it was clearly an AI generated image. I mean, really clearly. And I think people are wanting to find things like that, like this jerk who is, you know, smoking in a McDonald's, and they want to have that, and they're losing their thinking brain. And that's where I'm like, Look, guys, you have to realize so much can be aI generated now that you have to really question, like when you see, you know, and even stuff we saw this happening that was before the big AI was doing it. It's just like Nancy Pelosi, when that recording was of her slurring. All that was done is they slowed down her voice. She wasn't slurring. That was a, you know, recording that slowed down her voice to make her sound like that. That wasn't AI. So it's not that we haven't had this before. It's just that the amount. There's two things that are happening. The amount is increasing, like unbelievably, because it's so easy to do. And then the second thing is people are getting highly targeted, so they know who is going to afford this and get riled up about it, and that is AI. AI is doing that targeting where they're just going in. They're like, this person is gonna afford it to their 280 followers, but they have a million of those people that are doing that. Again,
Sean Lane 14:13
it bears repeating that Jana has been working with AI for decades, so while the AI hype has become more pervasive. It's not new. And Jan is saying that, in addition to the improvements to large language models, we're more aware of AI for two reasons. One, the volume of use cases is increasing, and two, people are being highly targeted by AI with AI driven content. All right, I want to shift gears away from some of these consumer use cases. And get back to AI in the B to B context. Given Jana work at narrow logics and with some of her customers, I wanted to understand what she sees companies getting wrong when it comes to using AI in their businesses. They
Jana Eggers 14:54
think it's magic, like I said, they're believing that the AI is smarter than. Them. And I'm not saying that it's not smarter. It's probably just seeing things differently. You know, I like to think of it kind of like as a prism or something, and it's like, Hey, I'm looking at it this way, and you're looking at this way. And it doesn't mean that that's not valid, and it could be interesting for some things, like, why does it do it that way, understanding that more but I think that this idea that, because the model is taking in more information, it's smarter than us, so it's reading all of the encyclopedias, so it gets it and it doesn't necessarily it's just a next word I mean, if you're talking about chatgpt, it's just a really smart next word generator. It's not understanding anything. That doesn't mean it can't come up with something creative at all. But it's us evaluating that, rather than it evaluating it. So it didn't go in and go, Oh, this is a good answer. I will give this answer. It's just like, This is what I was trained to do, so that's what I'm doing. So I think that's the biggest thing that people are getting wrong, that amount of data matters, the right type of data, how that data is curated, how that data is then ingested and used, and what the purpose of that was. One of my customers did this, which was totally cool. They wrote me, and they were like, Have you ever asked chatgpt to say, compare for me using Nara logic, synaptic intelligence platform, which is the name of our product, and chatgpt for decision making. And it came right out and said, I'm a language model. I don't make decisions. And it actually gave a really good result. Like, I was like, this is brilliant. Why did we ever
Sean Lane 16:40
think of this? What is it on marketing? And I honestly,
Jana Eggers 16:42
when he texted me and told me he did that, I was like, Oh no, and I rolled my eyes. And I was like, that's gonna give some bogus answer. It was actually really good. And I was impressed with it. It's so much so that we played around with it, and we're like, this is really good. And the funniest thing that happened there is it really talked about decisioning and reasoning. Didn't even talk about explainability. And I feel like if you go onto our website, you just see explainability is all over there. So it wasn't using it wasn't just comparing what it thinks about itself and what we think about ourselves. It was actually doing some pretty insightful stuff. And I was like, Hey, I feel really good about this. So
Sean Lane 17:22
I actually was looking at the website myself, and I loved the succinctness of some of the definitions that you all apply to help people to be able to have these conversations. And so you actually started to talk about this on your website. You say, AI is the ability to use computer power to acquire and apply knowledge and skills in new ways. AI augments human intelligence. It does not mimic it. And I think the point you were making about how the models are trained and the data you bring into that is critical. So how do we better understand either if we're using something like chatgpt, or we're creating something are on our own within our companies, how do we better understand the inputs that are going in to then help us understand and explain the outputs that come out. Yeah, there's
Jana Eggers 18:07
a couple of things and redirect me, if I'm please, going the wrong direction on this as far as what you think is useful. So one thing I talk to people about is a computer computes. So think about a calculator, seriously, but when I'm talking about you understand this, you've used a calculator. But when I'm talking about statistically generated it's just looking at order of words and figuring out in certain contexts. This is why parameters are important, because parameters give you context. What is the right order of words to put out there? You don't think that way at all. That is not even close. You have a lot more conceptualization. They don't have much. There's a little bit, but it's a very thin layer. This is when you talk about, like, what are the hidden nodes? And in the, you know, neural net, we haven't proven that there's any conceptualization there, maybe a little bit, and people are still trying to figure out, what are those nodes, but it's more. I just got the statistics right. Then I understood that when I talk about an animal, that animal could be a pet or not be a pet, just as an example, it doesn't have that kind of concept. Now, there may be things like wild animals versus pets. And there's things that look like intelligence of understanding that, but it's really just what gets correlated when something's a wild animal versus what gets correlated when something is a pet, right? So Wild Animals don't usually have leashes. Pets do. So a leash is going to be talked about more when you talk about a pet than it is a wild animal, just as a quick example. So you have to think about what it's really doing, and it's really just computing. Janet's
Sean Lane 19:47
advice here is simple. Think about where a computer can actually help you to compute. And she goes further in some of her writings to say that AI should be designed for subject matter. Experts rather than technical ones. So maybe if you're in your company and you're looking for ways to leverage this tool to arrive at the outcomes you want, starting with areas in which you are a subject matter expert, might make a lot of sense. I asked Jan if this was the right way to take her advice, and how does she go about coaching people to find the right use cases that will actually be helpful for them. One
Jana Eggers 20:23
quick example, and it comes from, you know, the software engineering side. I mentioned coding and Amazon just recently said Annie Jassy said, Hey, we just saved 250 million. I think it was with AI, you know, replacing software engineering tasks, and if you read it, so first of all, that's like, point oh 3% of their budget. So as a startup, you think about saving point oh three and kind of like, Okay, nice. I get that it's good for them, and I get that it will grow. So please, I'm not poo pooing that. But you have to understand perspective, because that sounds really big, but it is honestly very tiny for even them, who, by the way, are some of the world leading experts in this. So they're just now getting these tiny numbers. That was point oh 3% by the way, not 3% point oh 3% and it was point 4% depending on which budget you're looking at. So are you looking at operating costs versus R and D and so you can kind of mess around, but this is probably operating because the actual problem they were solving was software updates. So this is where I'm using this library. The library has a new version, and every engineer knows. They kind of go in, they press the button, they wait to compile correctly. Did it integrate correctly, what? Depending on how they're doing it, and then they run the tests, right? And so it's usually a 15 minute task or so, depending on how big it is and stuff. It can be five, it can be 35 but it's not a big task. This isn't an hour long task unless you're doing a whole bunch of them, and you're basically clicking a few buttons and hoping there's no problems, and running your fleet test and all of that. So that's what they saved. That's what they automated. That's helpful perspective. So that wasn't really coding, which is what most people think, that is happening there. It was really an automation task. And how much of AI that really was, it probably was some, but not a lot, but there were a lot of things that you wired together to get there. And again, I'm not inside Amazon. I just read I went through and was like, Okay, what did they really do? And it's like, oh, they're saving on the update times. I'm like, what did they mean by update time? You know, I peel that onion to really understand better. And again, I am not saying that that is not important. I mean, trust me, my engineers would love it. But is that gonna save me two 50 million? Not even close. Is it gonna save me point? Oh, 3% maybe. And I'm not sure I care that much about that right now. So look at the problems that are really going to have an impact on you. Understand. Where is it that you're struggling? Where do you have those subject matter experts? Where do you have people that you can really empower, like radiologists? Is an example that's often used with AI. So fascinating thing that I just learned a couple of weeks ago is that actually the number of radiologists have increased since we've had AI to help, and it's it's a dramatic increase. And why is that? Because we've now been able to increase the throughput of testing. So before, when we used to have to have a radiologist take more time, now they can take less time. There's a great example of a subject matter expert that was just empowered by AI, because the machine is doing the part that they used to get bleary eyed, and they, you know, they were tired and things like that. And now they have this machine going, Hey, look at this area right here. And that's great. And now they can provide some explainability. Like, yeah, that does look suspicious. The AI also helped with this. Needs to be retaken. This isn't good enough, right? So you don't even have a radiologist looking at it at that point. So that, why have radiologists increased when we've actually decreased the time in their job? It's because I've actually increased their workload. I've actually now can do more tests. I can find out then, rather than having to reschedule another appointment, because we find out after the radiologists read it, right then there's an AI saying that's not a good enough image. And so right then I can retake the thing, which means I get many, many more people in and therefore I now need more radiologists,
Sean Lane 24:43
if I'm a subject matter expert, and let's keep going with the radiologist example. How do I there's a lot of noise right now, right there's so much noise. How do I know when it's worth it to for me, my company, my product, whatever, to build. Something that is net new to add to that yield you're going to get out of a radiologist versus leveraging some of the other tools or models or whatever that might be already out there, like, that's a I mean, this is not a new problem. You know, build versus buy versus partner, whatever. How do I think about that if I truly believe I am a subject matter expert in a particular topic. I mean, it depends,
Jana Eggers 25:22
like we're here at Startup Week, right? So what I'd say is, hey, there's a lot of opportunity to start new businesses for that right, finding the right subject matter experts and saying, Hey, this is a tool I can sell to many businesses, a lot of businesses. It's probably not worth it for them. Really going in and building all of your own models that takes a lot of work and money and money and special expertise that you probably don't have. So I do think that a buy strategy is good. The question is, what you're buying. So I always talk to people about, hey, I'm honestly a build strategy, because you're building me into your product. So I'm more selling to people who are building large, complex systems, and they need me because I have to integrate in a special way. I'm more going to sell the Salesforce than sell to you using Salesforce. And so that's kind of the difference. So you have to think about where you are in that like, if I had a quick little app, and we've talked about, we have some ideas like that that we would package up, but it's hard for me to make that decision when I'm still doing you know, our software is six to seven figures. You're further upstream in this problem, further upstream in that problem. That doesn't mean that at some point I won't go, Hey, look, there is an opportunity. I know this one problem well, and I can package it in a certain way that I can integrate with. I'm just gonna say Salesforce, because it's a well known platform, and I can package that up. And, by the way, with that, rather than, you know, I'm definitely very hands on and close to my customers. But for that, I can do more of a Hey, anybody can just sign up and get this and run away with it, which is pretty cool. And again, it have to be something where explainability is really important there for us.
Sean Lane 27:11
And how do we think about so this is an interesting question. So I just had somebody on the show recently who was basically talking about how the end users, at the end of everything, we just described the Salesforce users who Salesforce users, they're gonna have a pretty wide variety of problems. And to your point, there might be some subject matter experts or unique data sets that can plug into Salesforce and solve problem A, so A different one can solve problem B. But as the end user, I don't care. I just want the answer. And so if Salesforce app a solves problem A for me. I'm gonna go back to them and be like, Look, I got problem b here. Can you just do this too, right? Instead of me now going out and getting B and C and D and adding those all on. So where do you think that's gonna go? Is Salesforce app a gonna end up being the one that, like, just keeps tacking on more and more use cases and subject matter expertise so that I can do everything I need to do there, or am I gonna end up the same way we are right now? Which is, I need to integrate 25 different apps into my stack that all happen to solve different problems leveraging AI, but it's really not the same.
Jana Eggers 28:15
Yeah. I mean, it's kind of gonna depend on how much you need that subject matter expertise, how much a and b really interact with each other. How separate are they? You know, we've dealt and lived in a world where things are pretty separate. I mean, I I'm constantly amazed at our customers, where supply chain and marketing won't even share data across and, you know, I'm working with very large enterprises. So it happens, their territorial doesn't happen as much in mid and small companies. But I think you got to think about those kinds of things. Is, are people going to share their data, you know, and does that matter? So if I'm an A and B, where the data doesn't matter, if it's shared and it's different people using it, and maybe there's a little bit of interaction, then that matters less. But if it really is something that's core and I mean, you've seen that Salesforce add stuff. I mean, they make irrelevant apps that are in their marketplace on a regular basis, right? Because they're trying to expand their market, so it's not a problem that we haven't seen before. And I think that that's going to work out the same or similarly, I shouldn't say the same. I do think what's different is that AI doesn't just require coding and algorithms. I should say it requires data. And so who has that data is the real challenge. And so you may be using a foundational model that's open source, but what is your specialty? What's really being added on that's going to be the biggest challenge for startups themselves. And then the question is going to become, okay, well, if you're on a platform like that, how much does Salesforce share with you, and rightfully share with you, meaning they have permission to do that, because I'm their customer, and if you know, I have. Data, then I'm able to customize whatever I'm doing with that. And so it's my basic point. There is it's much more complicated. Now we know how to do these things, but we don't know them at the level of complexity that we have been doing. I
Sean Lane 30:17
really like how Jana breaks down for us, the marketplace dynamics that are changing versus what's staying the same, according to her, while something like the Salesforce app exchange likely isn't going anywhere, the new wrinkle in all of these apps that are promising AI driven outcomes is data. Ai requires data. What's unique about your data that your app can bring to the table that others can the data on which these solutions are trained is the moat to differentiate one solution versus a sea of others. Which brings me to the least sexy word in all of these AI conversations, governance. Look, I know I don't really want to talk about it either, but your IT team is going to ask you about it, so you might as well be prepared. Janna, of course, sees the most rigorous version of this conversation through her work with her defense clients, but for the startup folks walking around at startup Boston week, I asked her how they should think about governance when it comes to AI, her answer wasn't what you might expect
Jana Eggers 31:18
in startups. It's really about your ethics, which overlays with your governance. So I'll give you a little bit of an example. So one of the companies that I ran was a company spread shirt still around, great company. It's kind of like Vistaprint more in Europe, but also more focused on clothing and higher quality and things like that. But for personalization, we allowed anybody to put whatever they wanted on a shirt. But there's a lot of things people will put on their shirts that all of us, well, I wouldn't say all this, some of us, most of us, would be like. And so we had a very open policy, and one of them was that anyone that was doing quality control could say, I don't approve of this. And we had a process that we would go through and talk about it together, and we would then go back to that person and say, Hey, we decided yes, we agree with you. Dog fighting isn't something that we're going to print, and we're going to do these things to curb that. Then we had other things that were copyrights, right? And Mickey Mouse ears is a good example. And so we had a lot of education too, where and not everybody knows every copyright we can't, right? And so, so there was a lot of education, there was a lot of communication. And one of the things that I'm very proud of that we did is because of that we actually would get and by the way, it was a German based company, and in Germany, basically you're guilty until proven innocent. I don't mean that in general, but in this kind of case, you basically get a fine, and then you have to prove that you don't have to pay that fine. And we paid very, very little, and it was all because we were very clear. We had a process that this wasn't something like, oh, we'll meet when we meet, and if something comes up, and it was, it was documented. We had minutes. We could show the people. We could show the judge. Hey, here's why, here's what we decided. Here are the things we talked about, and that's why we in general, didn't pay fines. That doesn't mean that we may have still made a decision and removed something off of our marketplace and things like that, but we had a process, and that's what people were more interested in. And so that's what I'd say, is, you know, have a process that follows your values. And our values were, don't infringe on copyrights. That's just uncool. We were more wanting to enable artists. I don't want artists stepping all over each other. And then the second thing is, support your people. If they're uncomfortable with something, let's figure out why, and then give them that feedback. And so those were our values, and we had very clear governance. And so as an example with that, you know, we work in defense and military, that's a sensitive subject, and so we talk in our team about it. We talk about it regularly. We tell people, Hey, if you have any issue, come and talk to the management team about it. We fully support you. We're not in a place where we can say, okay, we can have people that do that and people that don't. We all work on it. So it's something we're upfront about when we're recruiting, and it's something that we openly talk about as a team, and we also under it's like, if anything that a customer says makes you feel janky, talk to us about it, and let's talk through it and see where we are. I don't want our product used in an unethical way, but I also want us to help not make mistakes, which is where I think explainability is key, because if I say, for example, this is going to be targeted, I want to know all the reasons that tells me why that's. To be targeted. That's gonna be targeted whether they have my system in there or not, and that's just the reality. I wanna be in there. So I make sure we know the pedigree of that data, the provenance of that data, so that I can communicate more clearly, not just because the AI thinks that it's there. I wanna know what all led to that, and that's why I feel like for us, and for me personally, it's a responsibility, because I know AI can do this,
Sean Lane 35:29
I think, first of all, that is incredibly admirable and should be commended. And I also what I'm hearing from you is that the time you take to actually codify those values, talk about them, write them down, and then continue to reiterate them amongst your team. That's where they come to life and live and breathe. And I also think the really hard part about that it sounds like you all are living and breathing really well is the idea that there's no daylight between you and the rest of your leadership team on these things, because at the end of the day, these things have to come from the top down. You could have the best intentioned copyright infringement analyst person sitting there at their desk trying to do their gig, but if that's not something that's been prioritized by the organization and said out loud that this is the way we do it, and then they backed up through actions, then
Jana Eggers 36:15
doesn't matter. And by the way, it's hard. I mean, it takes effort. Yeah, it's not something that I can just say, Oh, my legal team is going to handle the copyright infringement. I mean, we could have done that, and we, I'm sure, and by the way, had an amazing legal team. They were brilliant, but they also knew that they were not the owners of it, of the process. They couldn't be. They were the guiders. They were the ones that helped us frame it, but they weren't the people that were sitting in quality control every day, right, right? They couldn't see what was actually happening. I mean, we could have put legal person there, but it wouldn't have been the best use of their time, right? So we had to really get people understanding why we were doing this and why it was important to us as a company, and we openly, by the way, also talked in the company about, hey, we got sued by these people. Not sued. We got fined through the process. But to me, as an American, so it's like, yeah, we sue everything. They would always laugh, you Americans. And I always told them. I was like, Look, we have bigger judgments, but you guys have more often judgments. I mean, that's the way. It's like, you're just used to $10,000 all the time, and we get these ten million every now and then. And we don't get the $10,000 all the time, but you had to get people involved in that and have them understand, no, we're not just making the right like this is happening all the time.
Sean Lane 37:42
You before we go, at the end of each show, we're going to ask each guest the same lightning round of questions. Ready? Here we go. Best book you've read in the last six months?
Jana Eggers 37:54
Oh, man, that's hard. I think you may have told me that you had asked me this, and I probably gave you a different answer. Then, because I love books, I'm really a believer in the anxious generation. So I love Jonathan, hate first of all, I think he's a fascinating author, and he wrote righteous mind, which is the first book that I read from him, but it's really the anxious generation is about. And I think every school teacher should read this, because school teachers have such a big impact on our kids lives. But this is really you know what screen time is doing to brains, and it's not just you'll learn for you and what the screen time is doing your brain, but also for your kids and what's really happening there, and in particular, as I am female, and what it's doing to young women is just traumatic and scary. And by the way, it's not just in the US, it's globally, and they have all the numbers to show that.
Sean Lane 38:51
I just bought that book. I haven't opened it yet, but I'm going to now, definitely you'll devour it. I promise. Normally ask favorite part about working in operations, your honorary here today. So favorite part about working with operations,
Jana Eggers 39:05
I feel like I'm in operations every day. You know, I've done so many startups, but I've never had the title of CEO. What I would say is, to me, operations just empowers everyone, like when you have a great operations person. They're the person that just is getting shit done right? You're like, cleaning up. It's like, you just make these amazing problems go away to where the rest of us are. Like, I didn't even understand how you do that. I don't get like, how you make sure these things happen. And I'll give you a tiny, trivial example, but this is what operations to me like, you know, just an example that someone said, another day, we had a conference room table that broke, and I was in a meeting, and I bought a conference room table while we were in the meeting, it broke and before the meeting, and. I had bought a conference room table that, to me, says everything about operations, like it's the people that just see the broken thing and fix it. And this person responded to me, she was like, I can't believe you just take care of stuff like that. I've never been in a company where things are just handled. And I thought about it for a minute and like, first of all, I'm insane, but secondly, it's really because you haven't been in a company that valued operations like that. And I don't, I'm just that's a trivial example, like I said, but I think it's exactly that kind of thing where people are looking for problems and making things operate,
Sean Lane 40:33
yeah, but it also shows you, like, and I've had this happen to me too, where, like, it's rare enough for people to take the time to remark on it right. Like, that thing that I told you everything I wanted you just did it right. Like, it's crazy anyways. Flip Side, least favorite part about working in OPS, I think that there's
Jana Eggers 40:50
a lot of people that don't realize that, is what I'd say the least. Like they don't realize I just made your life better. Like you were complaining about that, and I just made your life better. I think sometimes that happens because people don't tell you what their real problems are, you know, they don't really tell you their whole life. And so operations, people that are amazing are the ones that are asking, like, Okay, tell me about that again. And how do you do that? And I just actually upstairs gave an example of so Intuit does a lot of customer visits, and we were at a customer once, and they they're like, oh my gosh, that report, that's the because we were studying a certain area, that revenue report, oh my gosh, that's the most important report that we ever use. It really is like, Oh, you designed that. Oh, my God, whoa. I love that report. And then you go, Oh, cool. Can you show me, and they were like, oh, remember the most important report that they looked at every day? They didn't know the password to get on. They didn't know once we went and got the password. I mean, we didn't. They did. They got the password, they logged on. They didn't know how to navigate to get to it turns out it was a report that was printed out once a month, and they hardly looked at it. So people try and create things maybe around what you're asking, maybe because you had a bias and you win. And then ask them about this revenue report, then they're gonna love it. There's a lot of subtlety there, I would say. And so that's the worst part, is when people aren't excited about what you did, my guess is it's probably because you misunderstood what the real problem was, and that's not on you. I mean, they probably told you something different than really is their problem.
Sean Lane 42:29
We could do a whole episode just on that answer alone. Have
Jana Eggers 42:33
me back. Yeah, we'll cover that. I'll talk to you about at Intuit. I did over 500 customer visits myself over the years, mostly with small businesses. So I have many, many tales to tell.
Sean Lane 42:46
I would love that someone who impacted you getting to the job you have today. Oh, wow,
Jana Eggers 42:50
sorry. The thing that came to mind immediately is someone who's passed, and this weekend, I was cleaning out a storage space that I have, and I came across a letter from John Holland, who is really one of the founders of AI. He actually invented genetic algorithms. That's what he's best known for, but really understands AI. And I had met him. I was fortunate enough to meet him, and he was got engaged in my problem, and he just wrote me this brilliant letter that, honestly, I had forgotten about. But as I read it, I was like, This is why I do what I do, because someone like him asked me questions. He engaged with me. He was, you know, he was writing me, saying, I really think you need to go to graduate school for this. But here are these questions. And he said, as you can see, you know, this letter handwritten by someone brilliant, famous and all this. And he says, As you can see, I have more questions, so we must meet again soon. That's amazing. And that kind of impact, I'm not he's not the only one, but that kind of impact that you have on someone, not just giving your advice, but actually engaging with them, that has a huge impact. And I was so grateful that I found that letter and I didn't just throw it away because there were a lot of boxes. I was like, just gotta go. I'm not even gonna look. And I was like, but I can't, and I found that letter that was worth all the hours that I've spent on that, just to see that.
Sean Lane 44:26
Thank you for sharing that. That's an awesome story. All right, last one, one piece of advice for people who want to have your job someday, don't do it far, far away. Run. Don't do startups.
Jana Eggers 44:37
They're insane. Now, honestly, it's not all that it's cracked up to be. So really, think about what your motivation is. I have so many people say, Oh, you're a CEO. So you're the boss of everyone. I'm like, I am the boss of no one at all, not even my dog. Because really, if you're doing it right, your response. To your employees, you're responsible to your customers, you're responsible to your board. So if that's your motivation, you can find other ways of bossing people around that as much it's going to bring you much more joy. So I would say, if you want my job, start understanding how you understand customers. Find an area that you're really passionate about. I mean, again, reading that letter from John, it made me remember again, that I started out at Los so this is when I was at Los Alamos, and I started there. And when I would give answers to material scientists that were there as the computer person, they would say, but why? And so that's really what started me on my journey, and that always making the technology tell me, but why? Has been a big thread, not the only thread through my career, and it's one of those things where I backed up and said I'm where I'm supposed to be. So what is your you know, but why? Which is the explainability piece of what I'm doing, it doesn't always go direct. I didn't get to answer that at every place. So understand where your passion is. You don't always get to do what you're passionate about, but you can often find it. There's a lot more flexibility than people think in their roles. They don't have to do the role the way that
Sean Lane 46:22
the bullets on the job description Exactly, exactly. You
Jana Eggers 46:25
have a lot more flexibility. Now that takes courage, because people will put you down. And trust me, I've been put down. I'm a woman in tech. I've been put down a lot, but also I'm kind of weird, as you've probably guessed. And so there were plenty of people are like, because she just doesn't know. It's like, No, it's because I know more. And this is why. So you have to be okay with that too. When you're kind of creating your own job that may not be your thing, you may not want that too. And like realizing, no, actually, I just want to be good at this, rather than I want to be like, you know, pushing those boundaries and doing all that, my job is not one where you just say, Hey, I've got a clean desk, I've got an assistant. I'm just that kind of CEO. That's not me. So it depends on what you perceive as my job versus what is my job.
Sean Lane 47:26
Thanks so much to Jana for joining us on this week's episode of operations. Also special shout out to Stephanie Samantha and the entire crew at Boston Startup Week at Suffolk University for having us as a guest. If you liked what you heard from the show today, make sure you're subscribed so you get a new episode in your feed every other Friday. Also, if you learned something from Janet today, and how could you not, please leave us a review on Apple podcasts or wherever you get your podcast, six star reviews only. All right, that's gonna do it for me. Thanks so much for listening. We'll see you next
Unknown Speaker 47:56
time.