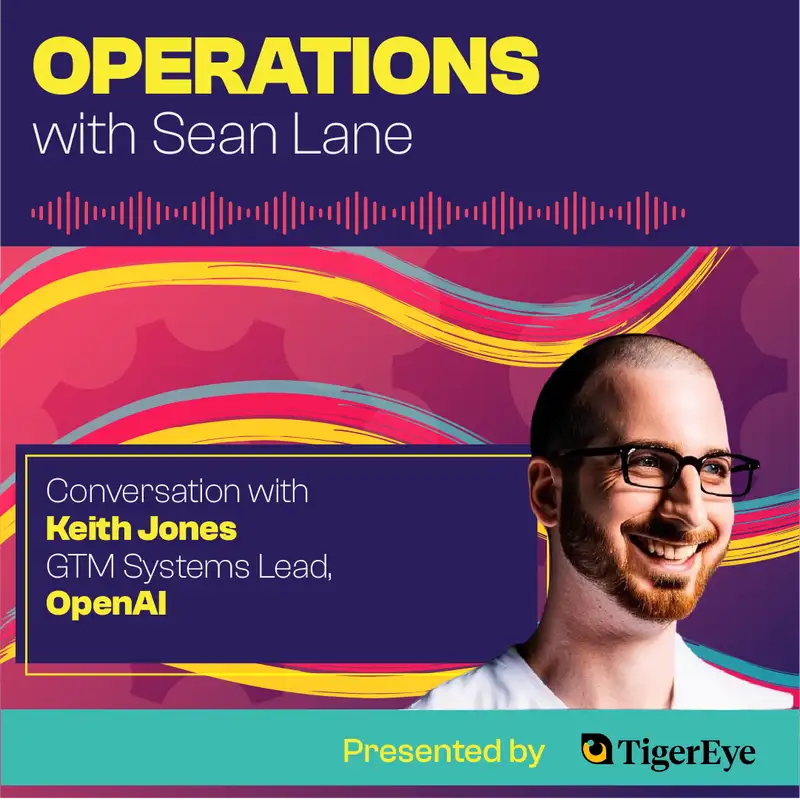
Inside OpenAI's Operations Team with Keith Jones
Sean Lane 0:01
listeners of this show know better than anybody that go to market is overtaxed and under resourced. tigereye can help. They bring revenue management, segmentation and planning all together in one platform. tigereye monitors your CRM to learn from every change and predict where every rep and every team will clock out. It's easy to see who needs help and how to get them back on track. tigereye extends your rep ops team with personalized AI to accelerate planning, segmentation and revenue growth. Go to Market is faster and smarter with Tiger eye, learn more and schedule a personalized demo at Tiger ai.com.
Hey, everyone, welcome to operations the show where we look under the hood of companies in hyper growth. My name is Shawn Lee. If you're having a conversation about the world's most innovative companies right now, it's hard to imagine a conversation that doesn't mention open AI. For the record Fast Company just ranked them ninth in the world on their 2024 list of the world's most innovative companies. Quick aside, they were edged out for the number eight on the list by Taco Bell. Anyway, open AI specifically open AI is chat GPT has opened up a whole new world of possibilities for everyone. But of course, on this show, we care about its impact on operators. So who better to talk to about this impact than someone who actually leads systems and operations at open AI? That's right. Today, we are going inside the walls of open AI itself with Keith Jones, the company's go to market systems lead. And while of course, I wanted to know what Keith is doing for his internal customers at open AI. I also wanted to be a little selfish today and learn how he's serving himself and the other ops folks within the company. In our conversation, we talk about a world in which teams spend less time inputting data into a CRM and more time conversing with it, we explore a very specific internal use case for a GPT that he built himself for himself at open AI, and how he makes the call of whether to use his own technology or not. To start though, I wanted to hear about the moment that Keith got to open it I in November of 2023, exactly one year after the initial release of chat GPT I figured that this had to be a pretty interesting time to join the orc.
Keith Jones 2:39
Interesting doesn't begin to describe the experience coming in, you know, for context, right November last year was about a year after chat GPT had been introduced to the world. And only a few short months after chat GPT enterprise was made available in a b2b fashion. So we were certainly kind of in a active learning state, if you will, are still in that state today. But what I would say in terms of the specific questions around what I thought AI was for ops then versus what I think it is now, I'd say first and foremost that, you know, I had a little bit of unique perspective, having come directly from Gartner, where thanks to the introduction and ripple effect of check UBT, almost the entire industry analysts population have been sort of re steered towards that to provide the market and Gardner's clients with insight on that. And we were starting to contextualize Gen AI alongside all the other types of AI. So I would say that the way I would describe my perspective at a time was that I knew I needed to understand where Gen AI came in versus where a different type of AI might already be applied. And I would say as ops, specifically, what I saw as one of my core responsibilities was to help my stakeholders understand how to tell the difference, so that when they were speaking about it, both internally and externally, we can make that clear to the respective audience. I think that's where things got kind of twisted, if you will, right, is everyone heard about chat TPT everyone heard the term AI attached to it. And then every vendor under the sun started to say AI this AI that we have aI when you and I both know that AI has been around a lot longer than chat GPT has been or at least longer than the world has known chat TBT I should say. And so I think that was really like my initial perspective. But then fast forwarding to today, I see it as a little bit more of a paradigm shift. So to add context, this I came up old school, right I taught myself how to use Salesforce and other salesmen. revenue technology tools. And I was a seller before I was a Salesforce admin. And so I had to use CRM before I managed CRM. And while that helped inform my opinion of how to create better user experiences, I've always leaned into, we have to account for every possible input, we have to try and proctor their experience as much as possible. And while that may not be the most fun experience, if it's straightforward, if it's predictable, if it's reliable, that's still better. And that meant validation rules and a lot of automations to go ahead and do these things. But at the end of the day, I was still completely reliant on the input from the seller. Right. And we designed CRMs, around what we expected sellers to see and hear on calls and put them in today. My perspective is that Gen AI specifically can play a role to remove the dependency on the seller for that direct input. That doesn't mean that we're not going to rely on the things they say in here. Absolutely we are. That's actually probably the crux of it, you know, in an increasingly digital sale, more and more organizations are falling into a norm of being able to record their calls and transcribe them and analyze them, whether it be with a gong or a chorus or another similar tool. And that's a lot of unstructured data that you capture at that point. But that's where Ji shines, is taking unstructured data and turning it into structured insight. And so and maybe
Sean Lane 6:43
just real quick, because you made that distinction for yourself and for your internal customers as you came into the business. For our audience, can you make that same distinction between the generative AI use cases that you're talking about now? And maybe some of those previous versions of AI that you were alluding to?
Keith Jones 7:00
Yeah, yeah. So again, some of the previous versions, like whether it was, you know, machine learning or like a predictive insight, right, it was all about kind of static analysis that you would prompt and provide to the seller, and they still had to do something with it. Right? What I would reframe Gen AI as is that we're doing something with what the seller is doing already, versus trying to get them to do something with an AI generated piece of information. So case in point, two really simple examples. lead scoring, that is, in its way, a form of AI, right, we're looking at pattern analysis and different data points to output something. And in an ideal world, the seller prioritizes, a lead with a higher score, that's a, you know, a form of predictive AI essentially, or at least hopefully, there's some sort of predictive model behind it in an ideal deployment. But that's, again, a data point where we're presenting a seller and we're hoping the seller does something with that data point. Whereas now with Gen AI, we can say sell or go do the thing that you know, you need to do. And we'll take what happened during that time and do something cool with it after the fact. So one of the goals that I have, personally, as a systems leader at open AI, is I want to get to what I'm currently calling like zero point CRM, I don't want sellers to have to manually input a majority of the information, because reality is most of what I need from them is going to be said heard, transcribed, analyzed, or otherwise collected, digitally. And if that is the case, which I believe it is, then I should be able to ingest that information, give it to an LLM and have it put it in a format that something like a Salesforce will accept. And if I can do that, now I can just say, go do the thing you need to do, maybe with that lead scoring, right. But then we'll do something on your behalf after you've done that thing. Zero
Sean Lane 8:54
point CRM, pretty compelling Northstar, right. I also love Keith's way of putting his approach with his sellers, you go do the things you need to do, we'll do something cool with it after the fact. I also do appreciate his educational approach to making distinctions between previously available forms of AI and the new generative form of AI that something like chat GPT offers to us. Let's pull that thread for a minute on those potential CRM values that AI might be able to capture instead of the reps. Historically, reps have had to fill out fields in their CRM to satisfy validation rules or to give a manager visibility into a deal for coaching purposes. So okay, even if the rep isn't responsible for doing that, that information is still valuable. So to Keith and his team, think about a shift in utility of what's actually being captured,
Keith Jones 9:46
what we're trying to achieve what that setup is to create more frequent opportunities, where a seller can make informed decisions and make them faster. So we're freeing them up some of the time cognitive load that they're having to put towards, did I put that billing email in correctly? Did I set the close date correctly? Did I add that contact to my opportunity, right? All this administrative load, like, we're trying to take it off of them, while getting that information still putting into the CRM, putting it somewhere where they can still see it and make use of it. So that's the part about being able to have the information they need. So they can make that informed decision and to make it more often and then make it faster. And by doing that, we're now creating more space for them to hone their craft, and to be human in the sales cycle. To your point, that's where the coaching comes in. That's where the advancement comes in. Because now, it's no longer a conversation of, well, I can't get to that deal, because I'm spending so much time updating my forecast. It's no, let's spend that extra hour like peeling apart that conversation and asking the LLM things that you said and did and what was really important and what wasn't so important in that call, because we actually have the time to do that now, as opposed to the 3040 50% of our time that we're spending doing admin work.
Sean Lane 11:12
And I think that last point is where it gets really compelling, right, which is not just how this information makes it into the CRM, but what you ultimately can then do with it, and not just for that particular deal, or that particular customer, but company wide or customer base wide. Because now you have the ability to look at those answers. Look at that qualitative information in aggregate, as opposed to just like okay, yeah, this information is going to help us win this individual deal. Am I thinking about that the right way?
Keith Jones 11:46
You are. And I would expand on it with a juxtaposition. Current state, I'm a sales rep, I need to figure out what am I going to do this week? Right? Obviously, I have my calendar at my inbox. I probably have a series of post it notes on my desk somewhere. Or maybe that's just me, I don't know. But I also probably have a Salesforce report, or several reports or tons of dashboards that I've been told to look at and see, you know, what deals so I have that are slipping, what deals do I have that haven't been updated in a little while, right? What if instead, I could just ask the CRM, which of my deals are set to close this week. And they could tell me that, if we can take unstructured data, turn it into structured data, we know that an LLM can retrieve information from structured data really well. Now, there's obviously finer configurations. And I'm glossing over the, you know, more specific details there intentionally. But the reality is that you can more than likely get to a point where the seller isn't having to spend time inputting data into CRM, and they can spend more time conversing with it, to get the information they need to make those informed decisions more often and faster. Okay,
Sean Lane 13:01
we're gonna come back to those more specific details a bit later, I promise. But just think about the possibilities of the world that Keith is describing. There's long been a promise by plenty of other tools for reps to spend less time on low quality activities, such as data input into the CRM, but this idea of conversing with your CRM, asking probing questions about your deals, your data, opens up a whole new type of possibility and thinking, and I for one, can't wait to see all of the new advancements that are still yet to come that operators will be able to use to make the lives of our internal customers better. But as I mentioned, at the top of the show, I want this conversation to be a little bit selfish for us as operators as well, I wanted to ask Keith, what has he learned since joining open AI that he can do to make his team's lives easier, his use case is gonna blow your way.
Keith Jones 13:56
So I have a particular use case that I'm seeing a lot of really interesting value from right now. And it's one that in my line of work comes up relatively often. So I'm pretty jazzed about the value I'm seeing now and how I can refine it going forward. But this is specific to evaluating vendors in the market. So I'm currently in a vendor evaluation. I'll spare you the details on the category of software on the vendors I'm looking at for the sake of the recording. But what I'll say is this, I have a lot of different stakeholders that have a vested interest in this evaluation. That's a really difficult thing to do to manage not only all of their opinions and desires and requirements out of a vendor in this evaluation, but also to manage the actual evaluation process, right? That's a lot of schedules to line up. That's a lot of information to disseminate to a different to a wider group. So I decided to tackle this with the help of Jack GPT and particularly Eygpt that I built for this evaluation, and I saw four through Two different avenues. The first is on the idea of managing sort of their initial requirements and what they need, right? Now, of course, I can go from person to person profile or profile from my sellers, to my folks, cross functional partners and other parts of the business who have this interest in this category of software. And I can do like interviews and like, ask for details, maybe I create a survey, right? That all is great and fine, but that takes time, and a lot of energy to. And so what I did instead is I created a really simple organic exercise, where I said, I'm going to ask you to take a blank Google Doc. And with a simple prompt of what do you need out of software X. And I'm going to put a time limit, I'm going to ask you instinctively just to dump out of your brain, like just type it out, don't worry about grammar, don't worry about it being super concise. Just give me the raw, even somewhat emotional reaction that you have to that, as it relates to your needs out of this particular software. I had them take one extra step though I had them then record themselves, narrating what they put on that Google Doc, because the reality is as humans, right, we do one thing, but then we see what we did. And we have additional thoughts and or completely different thoughts. So now I've got a Google doc of requirements, essentially, in loose form. But I also have contextual information in spoken form that can be transcribed and analyzed. And I gave both of those things to a GPT. And said, synthesize this and turn it into more concise level of requirements for the background of the person that I have. And I did that for every single person in our buying team. So I can present back to them, here's all your summaries and everything, versus me trying to structure it upfront, and force them into it, I allowed them to be who they are in their roles, while still coming out with a structured set of requirements at the end. So that's one particular way that's just at the front of the evaluation. Now throughout the evaluation have done something different is we've as we've met with different vendors, whether it's me one on one with them and discussing different aspects of the evaluation or about their services and their platform, or as inviting my colleagues into like demos and other conversations with those vendors, again, about their services and platforms, I've made a point to have every conversation recorded and transcribed and analyzed, and that what that allows me to do is then create a separate GPT that has all of that information, has access to the transcripts of those conversations, has very specific instructions, one first and foremost, to understand who are the finite list of vendors in this evaluation. Second, and this is a known, you know, limitation, or I should say, aspect of MLMs. Right, we all know, they can hallucinate from time to time. And so I specifically instructed this GPT to only ever search, it's knowledge that I've provided it. So it has a finite universe of things that it's been provided. It's a good, great bunch of detail, and a lot of unstructured data, because it's just a call transcript. Right? But that still knows to look there versus trying to come up with the answer on its own, right. And so what I ended up having is a GPT, that I could distribute to all the different members of my buying team and say, Hey, we're gonna have these meetings with these vendors. If you can make the meeting, please do. But if you can't know that, it'll be it'll be recorded. It'll be transcribed, it will be analyzed, and our GPT, which we decided to call evali. I don't know if that's the best name, but I'm sticking with it for now. They have access to it. And they can go in and ask it questions about what happened. The really cool part is because these are transcripts, we can actually have them ask specifically where in the call did that happen? Where in the call? Do we cover that topic or that feature or that piece of functionality? And then now they can go directly to the recording, and listen to that piece and see the raw recording for themselves. But how much faster? Are they able to do that? And also having not been in the room because of it? We're not done with this evaluation yet. And I've got lots of learnings already from it. But the feedback from the buying team has been fantastic. They were like this is such a cool application of our technology. I feel like I was actually there, even though it wasn't in the room during the demo, because I'm getting all the same information that I wouldn't have had it been there. Not
Sean Lane 19:43
to mention the fact that you're going to forget about stuff that happened during the evaluation later after you've already picked somebody you're going to come back and still be able to ask questions. Remember, they told us we could do this and we couldn't do this like so. That's great. So you've got one GPT for this kind of requirements gather During a separate one for the evaluations themselves, I would imagine at a certain point in this process, you're going to bring those two together. And then ultimately, how do you think about using everything you've built thus far to help you
Keith Jones 20:13
ultimately make a decision? Yeah. So there's one other component to this that I'm playing around with. So, you know, we took that somewhat more organic intake, right, we created a more structured rubric. And I have two dimensions to that rubric that I'm looking forward to kind of analyzing, if you will, and that is that one I have the vendors use that rubric to score themselves. But then I also have my stakeholders using the rubric, a blank version of it and scoring the vendors as we get further along and are spending more time with fewer vendors, because it's a lot to do with the full list. But about halfway through, we have fewer vendors, so they're scoring them. And so now I can start feeding the scorecards to them. So that there's a record of how well did you score against the requirements that we synthesized from your initial intake, right. And now when they're having conversations, or at least this would be the working theory, we're not at that stage, we're still in the middle of this evaluation, but that they can go in and say, How well does vendor x dou y, and what have me and my colleagues said about it, right? And then it's a helpful reminder of again, like, well, here's what they say that they do. Here's how well your colleagues scored them, here's how well you scored them. And here's how it compares against their own score. Right? This goes all the way back to that previous example the seller, right, all we're doing is making information more accessible, so they can make more informed decisions more often and faster. Because I promise you we are having really candid, extremely productive conversations about this evaluation internally. And we're doing it with very little like information gap with very little like, setup, I have an honest effort to really as much time facilitating the same value as I have previous ones in my career.
Sean Lane 22:07
When it comes to using medic and qualifying accurately, you need the data in your CRM, force management's opportunity manager or OM is now on the Salesforce AppExchange. O M medic captures key factors about sales opportunities, including customer pain points, metrics, relationships, and decision making details within the Salesforce opportunity record. Guided assessments help you qualify and advanced deals to closure. Customizable dashboards and reports help gauge organizational consistency. Check it out at force management.com/o M, that's force management.com/o. M. Okay, back to Keith. Before the break, Keith was breaking down for us a real life use case for building his own GPT. Within open AI, he and his team built evali. Which yes, if you're wondering is a play on the Pixar movies, Wally, to help them streamline and reduce the overall workload that comes with evaluating and selecting a vendor. If you've ever been the one that's responsible for all the requirements, gathering internal communications that come with picking a new tool, I'm sure you're about to go build one yourself based on case description. Which got me thinking, clearly evali is a value add for Keith, that makes part of his job easier.
Keith Jones 23:23
I mean, the hilarious part is that this is nothing new at this point. Now I'm I'm relying on all of the a lot of the things that those of us and ops and operators around the world know how to do almost instinctively, which is some sort of basic cost benefit analysis or impact versus effort or basic, just human pattern recognition of how often am I doing this darn thing, right? It's something I'm doing all the time. And I actually can cite another use case that I have, right? One thing that you know, I have to do on a regular basis is try to communicate to my stakeholders, and especially my immediate colleagues and revenue operations, what changes have we made to our Salesforce and to our systems over time, right? No surprises anyone, right? We have a project management tool, we're putting things in there, and we're updating them and closing them out. But I'm not going to ask, you know, a seller or a sales leader to go in and check every closed out task from that week of that month. But I am going to upload it to a GBT and say summarize this. Write me my release notes. Right? I've written more release notes than I care to remember. And for those that worked with me in previous companies know that I actually take pride in writing them and trying to make them fun and all of that, but I'd be lying if I said it doesn't take a ton of effort to make that happen. Now I can have that same quality product, but I can get it done in a fraction the amount of time again to the whole like cost benefit analysis or impact versus effort like that's something I have to do with consistency and on a fairly regular basis. So those are two sort of things in terms of what the need is and how often I'm doing it, that make it a key candidate for something that a GPT ought to help me do better and more often.
Sean Lane 25:10
And then if I kind of think through what comes next after that decision, the next questions, which again, are all the same questions we've been asking forever, but now just like the past two, those answers may have changed is alright, if this is my new means for dealing with this particular problem, the next question is going to be alright, well, how does this new means play nicely? Or not so nicely with all of my other systems and my other processes? How do I bring the same level of visibility and transparency, going back to your Salesforce example, where everyone's used to being able to see this stuff? And so if you're experimenting by building different TPTs, for these processes, but then ultimately, you still need to think about how this might interact with the rest of the company's data or with the rest of the end users in a company internally? How do you think about that, right? Like, and you're living and breathing, the frontlines of this? And so you're, I think you're a great person to ask about this, like, does all of that then stay in GPT keys that get shared with people? Do you think about tying them back into like other systems of record or sources of truth? Like, help us kind of break that problem down?
Keith Jones 26:21
No, that's a great question. And I think I, you know, I'll preface this by saying that I have a pretty specific opinion as a systems leader, not necessarily as an open AI staff member, right. But the reality is that data security has to be of the utmost concern right. Now, thankfully, chat, GBT enterprise and our services have all the proper security measures in place. But the reality is that that data shouldn't be stored in the GPT, and should be able to access the data where it's stored elsewhere, also securely, but also where it's being updated by whatever sources, it's being derived from on a regular basis, right. So case, in point, the first like prototype of that of the value that I was building was based off of static data that I was uploading it to, right, but a future state was able to ping where the call is stored, and access it in real time. And that's really what we should be marching towards, because you don't want to have these points of failure that exists where the data is being replicated and stored in multiple places. And it's interesting to note that you asked, I was just perusing LinkedIn earlier this morning, you know, as you do, and I saw a piece about how MLMs were like, we're going to be the death of the CRM. And I actually took a little bit of issue with that, because, to me, it's not so much that the LM is ability to provide analysis of unstructured data means we don't need structured data anymore. It just means that who is responsible for ensuring that data is structured, or maybe more accurately, what is responsible for ensuring that data is structured, that's what's shifting. And now we can do more cool things with that data structured or unstructured, thanks to the LLM. And the LM can handle some of the transformation from unstructured to structured but we still need structured data, because you still need certain assurances, you still need to be able to have certain controls and compliance obligations have to be met. And so one of the things that I think is, you know, we have to acknowledge is the nature of this technology, and our use of it will only advance as fast as our society's ability to understand it, and adapt to it. So one of the points I was making when reading about, you know, will this kill the CRM is I was like, Well, no, it's not going to because for as long as we know, we are still going to be subject to various regulations and other compliance matters. And those aren't going away anytime soon. Governments and Governmental bodies are not going to stop asking for certain things, right. So Dave is still going to need to be structured somewhere, it just means that we will have less dependency on ourselves to make it structured, which means we can go do other things with our time.
Sean Lane 29:22
So prognostications about the death of the CRM aside, what I took away from Keith here is that there are still some foundational things that we're used to that won't necessarily be going away anytime soon. You still need structured data, you absolutely still need to care about data security and compliance. And then on top of that, the quote, cool things he talks about, that we all get to do is I think what gets a lot of people excited. And unless you work at open AI, or you have someone like Keith at your disposal, it might be really difficult to stay on top of what's possible and what use cases are out there. So how does Keith himself I'll learn and stay ahead of the curve.
Keith Jones 30:02
So funny enough, and this might sound counterintuitive, but I actually have a particular called source of inspiration, if you will, that I prefer for these types of situations. And that is specifically someone who's not technically minded. I'll actually give you an example that, you know, gave me an idea. And it was something I latched on to and we're slowly but surely kind of piloting it out. But, you know, early on in my tenure here, I was rolling out a new piece of software, you know, we're, you know, we're building our go to market org up, and we're bringing on new pieces of tooling. And it's, we're hosting this training, and someone, you know, lots of good questions are coming up, and someone just like raises their hand and they were like, can we just have a GPT? To only answer our questions about this software? And it's like, of course, we can, like why wouldn't we? Right? It just requires the right refinement and the right, I've got to be listening to that, right. So the moral story is this, you can be the most technically minded person, you can be the most operationally minded person, but until you are on the front line, in a sales organization, or go to market or division, finance, whatever, like, you shouldn't be talking to the people who are doing the job every day that you are supporting, and just listen to what I crazy ideas that they might come up with. Because even if you can only do 50% of their crazy, high lofty idea that's still 50%, or something that didn't exist beforehand. So that's where I go first is to the field, tell me what kind of almost science fiction type things come to mind. And I'll see what we can make possible. I
Sean Lane 31:39
also think that the thought you put into the answers you get back to that question will continue to be just as important as it was before the concept of a GPT was even possible, right? Because just like any other technologies or tools, like, you know, if you wake up a year from now, and you've got 50 GP T's running, that's just as helpful as having zero. You know what I mean? Like, for those frontline end users, they're not going to jump around between your 50 different ones, right, you still have to craft that end user experience in a way that makes their day in the life easier.
Keith Jones 32:18
Yeah, no, I mean, you're absolutely right. Probably the hardest part about this is figuring out what is too much what is too little, when they go scalpel, when they go hammer, right. But you've got to design the use of these tools around their day to day lives. Right. And sometimes less is more. I mean, the answer might be that you don't need another GBT. But you need to go back and revisit an existing one, right? Or it might be that you haven't positioned that GPT well enough, or socialized it as well as you could have, or maybe made it as accessible, right? Because there's a very big difference between me having a library of GPT is whether it's natively inside GPT or stored in a Atlassian page, like in Confluence or notion or something like that, versus me making a GPT accessible in the context of what they're doing every day. Right? I'd rather you know, be able to prompt them and say, Hey, do you need help with this? Click here. And then that takes them to the GPT? Or brings the GPT to where they are, right? I think those are the finer points that we as operators need to identify. But we also need to listen to our stakeholders and ask Where would this actually be helpful? versus where am I forcing you to think about something that doesn't occur to you naturally.
Sean Lane 33:41
And as you start to stack up more and more of those use cases? Right? And I know you're also thinking really thoughtfully about how to build your team? Like, how are you thinking about what that will look like, from a roles and responsibilities standpoint, as more people join the team, as more of those use cases get stacked on top of each other? Like, have you figured out yet kind of what that assignment of responsibilities might look like? And then, you know, maybe as a potential follow up to that, like, I would have to imagine some of the older ratios that we've used to determine headcount of off people versus the people we're supporting, are also going to be challenged quite a bit as we're going through this shift.
Keith Jones 34:25
Yeah, I mean, I can answer that question more specifically through the lens of go to market systems. Right and less true, you know, Rob offices general, just because I've been, you know, more in the in the systems world within Reb ops for the last, you know, five or six years, but what I would say is this, and maybe we'll have to have a follow up conversation, are they gonna be shot and see if it worked out or not, but I took a very different angle this time around. And in terms of headcount, and roles and responsibilities and tasks. I chose to hire product managers first, before I hire technical Will people. The reason for that is because I think a lot of the technical stuff that we could solve before with internal tooling or functionality is available from the user like there's, there are a lot of use cases that can be had and done through the use of this technology, that opening is brought to the world and that others are working on now. And I find it more valuable to have people who know the technology well enough to be informed and be a domain expert on it. But also tactful and artful enough to be able to have good conversations with our stakeholders, and bridge the gap between the two. Right. So I think the other sort of thought process here is that the technical work ebbs and flows, no matter how much I want to acknowledge that like I know that my function has, has value to bring, but like, there are weeks where we do lots of really heavy stuff. And there are weeks where we do really minor things, right. And so one of the things I'm doing is the the more technical like configuration style work. I'm outsourcing it right now. And that's because I don't have a consistent pattern for it just yet. But what I do have a consistent need for is understanding the requirements of my stakeholders. And so I chose to hire those people. First, we'll see how it works out.
Sean Lane 36:25
Before we go, at the end of each show, we're going to ask each guest the same lightning round of questions. Ready. Here we go. Best book you've read in the last six months,
Keith Jones 36:36
I'm not gonna say the full title out loud, because it's got an expletive in it. But it's both personal and thought provoking. But the title is, everyone you know, is going to die. And it's by a comedian of all people, by Daniel sloths. But I love the book, because he's just very blunt, but also offers very cerebral sort of comedic takes on various aspects of life, from familial relationships, to romantic relationships, all in the sort of general context of like, we're all not going to be here forever. So let's make the most of it. And I find it really refreshing. I love it. I love it. My
Sean Lane 37:17
favorite part about working in OPS,
Keith Jones 37:19
I think my favorite part about working in OPS is that in this is going to be a really selfish answer. And it's really the truth of why I'm an ops and I'm not in other roles, I get to be close enough to the action, to see it happen to see the value that wherever I'm working what they bring to the customer. But I don't actually have to do that part. I have the utmost respect for the folks in sales and technical and customer success I work with who are trying to manage expectations and direct their customers in various ways and everything else. And I know that I am not as good at that as I am at this. So I love being an ops because I get to help those people. And I get to, you know, take part in their success a little bit. But I don't actually have to do the parts of the job that frankly, I'm not very good at I would classify that as a self aware, not necessarily selfish answer. But I was saying I was in sales for a little while. And I was good at certain aspects of the job. But if you'd asked me to cold call anyone, you were barking up the wrong tree.
Sean Lane 38:25
Flipside least favorite part about working in OPS,
Keith Jones 38:27
managing expectations, it's both an internal and external challenge. Its internal, because I want to make everyone happy. I want to be that, you know, enabler of good things and good work. But it also means that I have to be really decisive about what I say yes to, when I say no to how I say no to it. And I hate doing it. Like I hate me having to say like, say no to things. But the reality is that that's probably one of the most important aspects of my job is saying no, and saying it in a particular way.
Sean Lane 39:01
Someone who impacted you get into the job you have today, someone whose job was I got
Keith Jones 39:05
today, I think one person that comes to mind is a former colleague of mine who still works at Gartner. His name is Dan Gottlieb. He is an analyst there. He was instrumental in like my onboarding at Gartner in between sort of startup gigs and open AI. But he also taught me a lot of how to be more open minded in terms of my analysis of a current situation and what to look for what to ask. I like to think that he like he taught me how to be an analyst. And that job prompted me perfectly for coming into open AI where I needed to have a much more open mind because of the actual nature of this technology. I couldn't just do everything the same way. I've done it before. I had to think about how to do it differently. That
Sean Lane 39:47
sounds like a whole nother episode in and of itself that that answer. Alright, last one, one piece of advice for people who want to have your job someday.
Keith Jones 39:55
I think one piece of advice that I would give to people who want to have my job is to figure out how to be as consistent as you can in certain areas and how to say I can't do that thing right now, that's a little bit different than the earlier answer about like me saying no to people, because when I said that I'm referring to like, we can't do that that's a request, we will not be able to meet right, versus saying I can't do this right now. Because the reality is that I think that's one of the hardest parts of my role in particular, is managing expectations and managing time commitments. So I would say, just learn how to do that. And to do it well, and learn how to under promise and over deliver, rather be in that situation than in the inverse. Yeah,
Sean Lane 40:48
I think that's fascinating. I think we've heard you know, different versions or iterations of similar advice. But I think your core point about consistency, we might not have heard from somebody before. And I think it's just like such an important thing for people that if you are consistent in the way you show up in the way you work in the way you deliver, over and over and over again, that is among the best value you can bring to the gig. Absolutely.
Keith Jones 41:16
I mean, it also it creates, you know, a reputation for yourself that they know they can rely on, even if they think they have to go into a conversation and tempting you, to convince you of something that maybe you're not thinking at the time. They understand how you work, they understand how you operate, and so they're willing to anticipate that right? I think I would add on ever so slightly, though, and I would say also like learn how to work with other people and not try to do it all yourself. I was speaking to a candidate. And I was asking about, you know, how do you navigate competing priorities? Because that's another hard part about this job and setting those expectations, right. And their response was almost immediate and started with, well, prioritization is a team effort. And I like Absolutely, it is not a decision you make. It is a decision that you all make together. And you have to be able to bring the right people in the room to be able to do that effectively. So I would say also, bring the people in the room don't try to silo the decision making.
Sean Lane 42:20
Thanks so much to Keith for joining us on this episode of operations and giving us an inside look into what happens inside of open AI and his role there. If you'd like we heard today, make sure subscribe to our show new episode comes out every other Friday. Also, if you learn something from Keith today or from any of our episodes, make sure you leave us a review on Apple podcasts or wherever you get your podcasts. It really helps folks to find the show and of course, six star review. Alright, that's gonna do it for me. Thanks so much for listening.